We Now Know How AI "Thinks"—and It's Barely Thinking At All
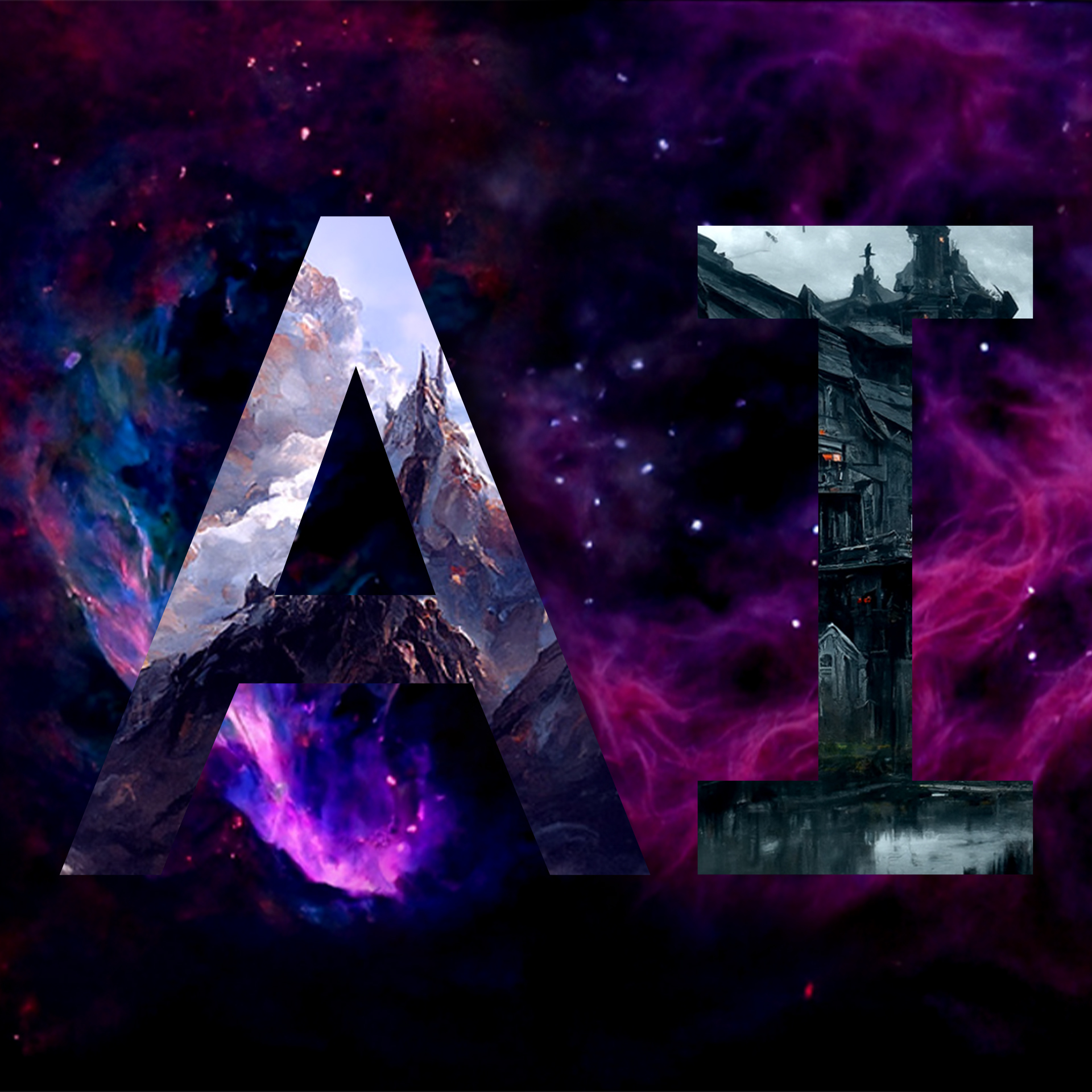
Table of Contents
The Illusion of Intelligence: AI's Statistical Approach
The impressive capabilities of modern AI often create an illusion of intelligence. However, a closer look reveals that AI thinking relies primarily on identifying statistical correlations within vast datasets, rather than genuine comprehension.
Statistical Correlations, Not Understanding
- Example: An AI can identify a cat in an image based on the statistical likelihood of certain pixel patterns occurring together. This is not because it "understands" what a cat is, but because it has learned to associate specific visual features with the label "cat" through exposure to millions of labeled images. This approach to AI thinking highlights a critical difference between human and artificial intelligence.
- Limitations: This reliance on statistical correlations leads to significant limitations. AI struggles to generalize its knowledge to unseen scenarios, often failing spectacularly when presented with situations that deviate from its training data. The system's decision-making process is often opaque, hence the term "black box" AI – we see the input and output but lack understanding of the internal processes.
- Bias in Training Data: Another significant issue is the susceptibility of AI to bias present within the training data. If the data used to train an AI is biased, the AI will likely perpetuate and even amplify those biases in its outputs, leading to unfair or discriminatory outcomes.
The Role of Big Data
The success of current AI systems is intrinsically linked to the availability of massive datasets. This dependence highlights the fact that the intelligence is not inherent to the algorithm itself, but rather resides in the data used to train it.
- Data Doesn't Equal Understanding: Increased data volume doesn't automatically translate to increased understanding. An AI might be able to accurately predict certain outcomes based on statistical patterns in data, but this doesn't imply that it grasps the underlying causal mechanisms.
- Ethical Concerns: The reliance on massive datasets raises ethical concerns related to data privacy, bias, and potential misuse. The energy consumption associated with training these large models is also environmentally problematic.
- Environmental Impact: Training sophisticated AI models often requires significant computational resources, leading to substantial energy consumption and a large carbon footprint. This environmental cost is a crucial consideration in evaluating the sustainability of current AI development.
Lack of Common Sense and Reasoning
Despite impressive achievements, current AI systems demonstrate a significant lack of common sense and reasoning abilities—a stark contrast to human intelligence.
The Absence of Contextual Understanding
AI struggles with nuanced situations that require contextual understanding and common sense reasoning.
- AI Failures: Numerous examples illustrate AI's failure to apply common sense reasoning. Image recognition systems might misclassify an image due to an unusual perspective or lighting conditions. Chatbots can generate nonsensical or contradictory responses because they lack a comprehensive understanding of the conversation context.
- Limited Knowledge Transfer: Current AI systems typically lack the ability to apply knowledge learned in one domain to another. A system trained to recognize objects in images might not be able to use that knowledge to understand descriptions of those objects in text.
- Rule-Based Limitations: Many AI systems rely on predefined rules and algorithms, which are inflexible and struggle to adapt to unexpected or unusual situations. This rigid structure is a major limitation compared to the flexible and adaptive nature of human reasoning.
The Challenge of Explainability
Another major hurdle in understanding AI thinking is the difficulty in explaining how complex AI models arrive at their conclusions.
- Explainable AI (XAI): The development of explainable AI (XAI) is crucial for building trust, ensuring transparency, and addressing ethical concerns. Understanding how an AI reaches a decision allows us to identify potential biases and ensure fairness.
- Ongoing Research: Significant research is dedicated to developing more transparent AI systems, but current techniques still have significant limitations. Fully explaining the reasoning behind complex deep learning models remains a challenging open problem.
- Limitations of XAI: Current XAI techniques often provide simplified explanations that may not fully capture the intricacies of the AI's decision-making process.
The Future of AI Thinking: Towards True Intelligence?
The current state of AI thinking leaves much room for improvement. Future advancements need to move beyond simple statistical correlations to achieve true artificial intelligence.
Beyond Statistical Correlation
Future directions in AI research should focus on developing systems that can truly understand and reason, rather than just identify statistical patterns.
- Neuromorphic Computing: Neuromorphic computing, which mimics the structure and function of the human brain, holds potential for creating more intelligent and adaptable AI systems.
- Embodied AI: Embodied AI, which involves integrating AI systems with physical bodies, might improve contextual understanding and common sense reasoning by allowing the AI to interact with the world directly.
- Artificial General Intelligence (AGI): The ultimate goal for many AI researchers is to achieve Artificial General Intelligence (AGI) – an AI that possesses human-level intelligence and can perform a wide range of tasks. However, creating AGI presents enormous technical and ethical challenges.
Ethical Implications
The development of increasingly powerful AI systems raises significant ethical considerations.
- Responsible AI Development: Responsible AI development and deployment are paramount to mitigating potential risks and ensuring the beneficial use of this technology. This includes addressing issues such as bias, fairness, transparency, and accountability.
- Regulations and Guidelines: The need for appropriate regulations and guidelines to govern the development and use of AI is increasingly recognized. These regulations should address potential risks and ensure that AI is used ethically and responsibly.
- Societal Impact: The widespread adoption of AI will have a profound impact on society, affecting employment, social structures, and individual lives. It's critical to consider these societal impacts and strive for equitable outcomes.
Conclusion
In conclusion, while current AI systems can achieve impressive results, their "thinking" primarily relies on identifying statistical correlations within massive datasets. This approach to AI thinking lacks genuine understanding, common sense reasoning, and contextual awareness. Understanding the limitations of current AI is crucial. Continue exploring this fascinating field to stay informed about the ongoing development of AI and its profound implications for the future. The quest for true AI thinking requires a shift towards more robust, explainable, and ethically sound systems.
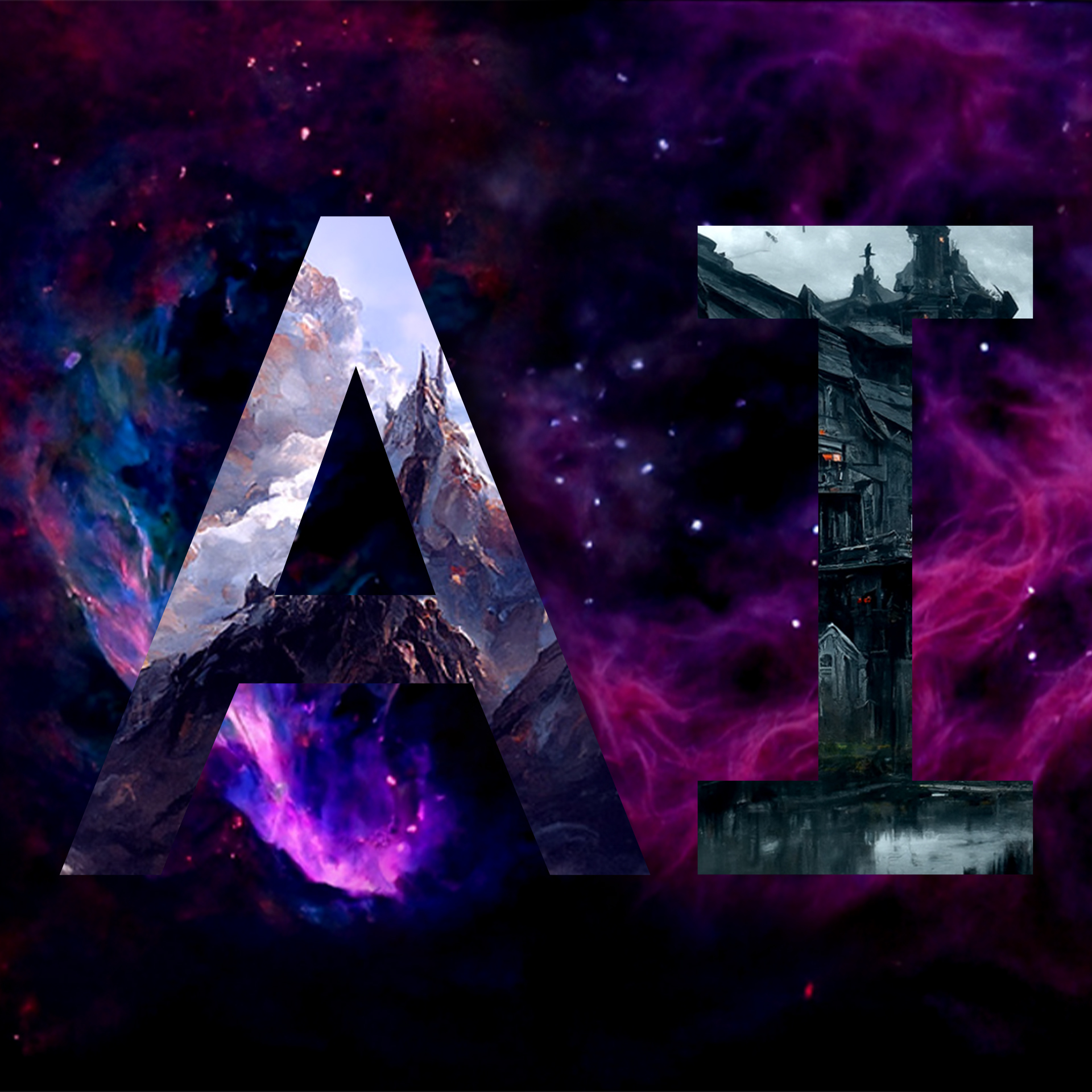
Featured Posts
-
Ray Epps Sues Fox News For Defamation Jan 6 Falsehoods Allegations
Apr 29, 2025 -
Georgia Deputies Shot During Traffic Stop One Killed Another Injured
Apr 29, 2025 -
Us Attorney General Demands Minnesota Enforce Transgender Athlete Ban
Apr 29, 2025 -
Are High Stock Valuations A Worry Bof As Analysis For Investors
Apr 29, 2025 -
Ignoring High Stock Valuations Bof As Rationale For Investors
Apr 29, 2025
Latest Posts
-
Millions Lost Office365 Executive Email Accounts Targeted In Major Data Breach
Apr 29, 2025 -
Inside Job Millions Stolen Via Office365 Executive Account Hacks
Apr 29, 2025 -
Cybercriminal Accused Of Millions In Office365 Executive Email Theft
Apr 29, 2025 -
Federal Charges Filed Millions Stolen Through Executive Office365 Compromises
Apr 29, 2025 -
Office365 Data Breach Millions Stolen Suspect Arrested
Apr 29, 2025