AI's Limited Thinking: What The Latest Research Reveals
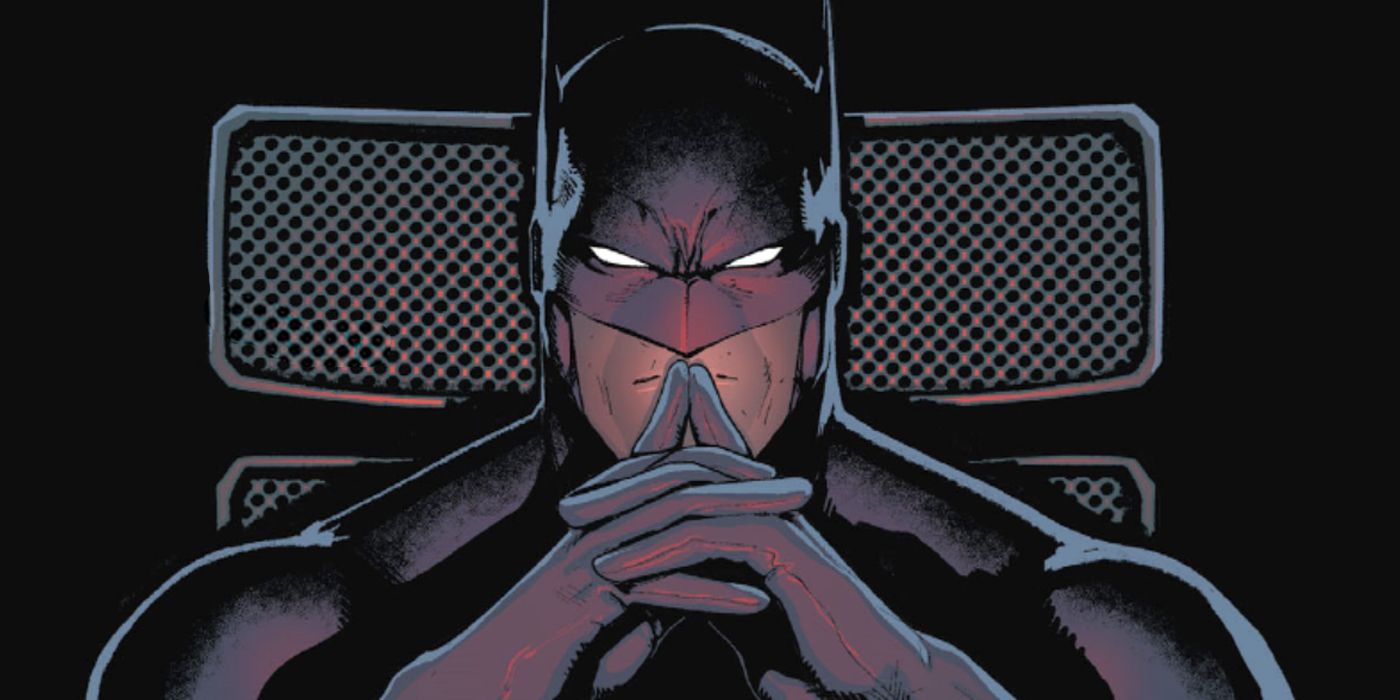
Table of Contents
H2: The Lack of Common Sense Reasoning in AI
Common sense reasoning—the ability to understand and apply everyday knowledge and make inferences based on implicit information—is crucial for intelligent behavior. Humans effortlessly navigate the complexities of the world using common sense, but AI struggles immensely. This lack of common sense is a major obstacle to creating truly intelligent machines.
- AI struggles with tasks requiring real-world knowledge and understanding. While AI excels at specific, well-defined tasks, it often fails when faced with ambiguous or nuanced situations. For example, an AI might correctly identify objects in an image but fail to understand the context or relationship between them.
- Examples of AI failures due to lack of common sense abound. Image recognition systems can misinterpret images due to a lack of contextual understanding. Natural language processing systems often struggle with sarcasm, irony, or implicit meanings. These failures highlight the significant gap between AI's capabilities and human-like intelligence.
- Programming common sense into AI systems is a formidable challenge. Unlike explicit rules, common sense relies on implicit knowledge and understanding, which is difficult to codify and represent computationally. Researchers are exploring various approaches, including knowledge graphs and symbolic AI, to address this limitation.
- Ongoing research focuses on incorporating large knowledge bases and reasoning mechanisms. Knowledge graphs aim to represent real-world knowledge in a structured format that AI can access and reason with. Symbolic AI methods, which use logical rules and symbols, offer another promising avenue for enhancing AI's common sense reasoning abilities.
H2: The Problem of Generalization and Transfer Learning
Generalization and transfer learning are key aspects of human intelligence. Generalization refers to the ability to apply learned knowledge to new, unseen situations. Transfer learning involves applying knowledge gained from one task to another related task. AI, particularly deep learning models, often struggles with both.
- AI models often perform poorly when faced with situations outside their training data. Deep learning models, despite their impressive performance on specific tasks, tend to be brittle and fail when presented with data that differs significantly from their training data. This is known as the generalization problem.
- The limitations of deep learning in generalizing knowledge to new contexts are well-documented. Deep learning models are data-hungry and require massive datasets for training. They often fail to generalize to situations not encountered during training, limiting their applicability in real-world scenarios.
- AI's failure to transfer learning from one task to another is another significant limitation. For instance, an AI trained to recognize cats might not be able to recognize dogs, even though both are animals. This contrasts with human learning, where knowledge readily transfers between related domains.
- Potential solutions, such as meta-learning and few-shot learning, are being actively researched. Meta-learning aims to learn how to learn, enabling AI to adapt more quickly to new tasks. Few-shot learning focuses on enabling AI to learn from limited data, thereby improving its ability to generalize.
H2: The Absence of True Understanding and Consciousness
A critical limitation of current AI systems is the absence of true understanding and consciousness. While AI can process information and perform complex tasks, it lacks the capacity for genuine comprehension and subjective experience.
- AI's ability to process information is distinct from its capacity for true understanding. AI systems can manipulate symbols and patterns, but this doesn't equate to understanding the meaning or significance of that information. They can mimic human language and behavior without possessing the underlying understanding.
- The concept of consciousness remains a mystery, and its absence in AI is a significant constraint. Consciousness refers to subjective experience and awareness, a quality currently absent in AI systems. Creating conscious AI is a highly ambitious and potentially controversial goal.
- The ethical implications of creating AI that mimics human intelligence without understanding are profound. AI systems could make decisions with far-reaching consequences without truly understanding the implications of their actions. This necessitates careful consideration of ethical guidelines and safeguards.
- The ongoing debate about the possibility of achieving conscious AI highlights the fundamental limitations of current approaches. Some researchers believe conscious AI is possible, while others argue it may be fundamentally impossible to create artificial consciousness.
H2: Bias and Ethical Considerations in AI's Limited Thinking
AI systems are susceptible to bias, reflecting the biases present in the data they are trained on. This can lead to unfair or discriminatory outcomes in various applications.
- Examples of AI bias are prevalent in areas like facial recognition, loan applications, and criminal justice. Facial recognition systems have shown biases against certain ethnic groups, loan application algorithms have discriminated against specific demographic groups, and AI-driven criminal justice tools have perpetuated existing inequalities.
- These biases perpetuate societal inequalities and reinforce existing prejudices. AI systems, if not carefully designed and monitored, can amplify and exacerbate existing societal biases, leading to unfair and discriminatory outcomes.
- Addressing bias in AI development and deployment is crucial for ensuring fairness and equity. This requires careful data curation, the development of bias-mitigation techniques, and ongoing monitoring of AI systems for biases.
- Techniques for mitigating bias in AI systems include data augmentation, fairness-aware algorithms, and algorithmic auditing. Data augmentation involves increasing the diversity of training data. Fairness-aware algorithms are designed to minimize bias in their decision-making processes. Algorithmic auditing involves systematically evaluating AI systems for bias and unfairness.
3. Conclusion
This exploration of AI's limited thinking reveals significant challenges in creating truly intelligent machines. The lack of common sense reasoning, the difficulty of generalization and transfer learning, the absence of true understanding and consciousness, and the pervasive issue of bias all highlight the limitations of current AI technology. While significant progress has been made, these limitations underscore the need for continued research and development. The future of AI depends on addressing these challenges to ensure that AI systems are not only powerful but also ethical and beneficial to humanity. Understanding AI's limited thinking is crucial for its responsible development. Continue learning about the challenges and opportunities in this field to ensure that AI serves humanity ethically and effectively.
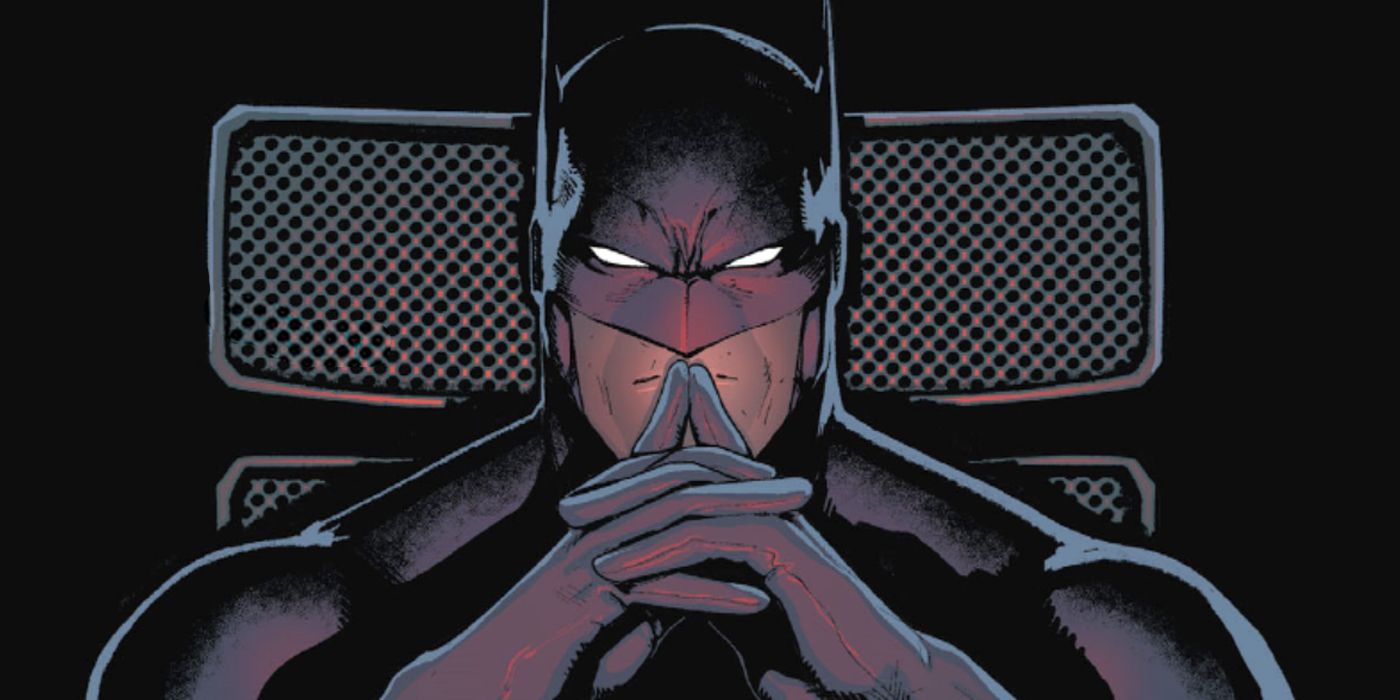
Featured Posts
-
The Complexities Of All American Manufacturing
Apr 29, 2025 -
Exclusive Huaweis Ai Chipset Aims To Disrupt The Global Market
Apr 29, 2025 -
From Humble Beginnings The Story Of Macario Martinezs Rise To National Prominence
Apr 29, 2025 -
The Legacy Of Murder An Ohio Doctors Parole Hearing And A Sons Journey
Apr 29, 2025 -
Ryujinx Emulator Development Halted Nintendos Involvement Explained
Apr 29, 2025
Latest Posts
-
Hudsons Bay Closing Sales Event 70 Off Final Markdown
Apr 29, 2025 -
Contempt Of Parliament Yukon Politicians Confront Mine Manager
Apr 29, 2025 -
The U S Dollars Trajectory A Historical Perspective On Presidential Economic Impacts
Apr 29, 2025 -
Hudsons Bay Liquidation Sale Up To 70 Off At Closing Stores
Apr 29, 2025 -
Yukon Mine Manager Faces Contempt Charges After Refusal To Testify
Apr 29, 2025