AI-Driven Podcast Creation: Analyzing And Transforming Repetitive Scatological Data
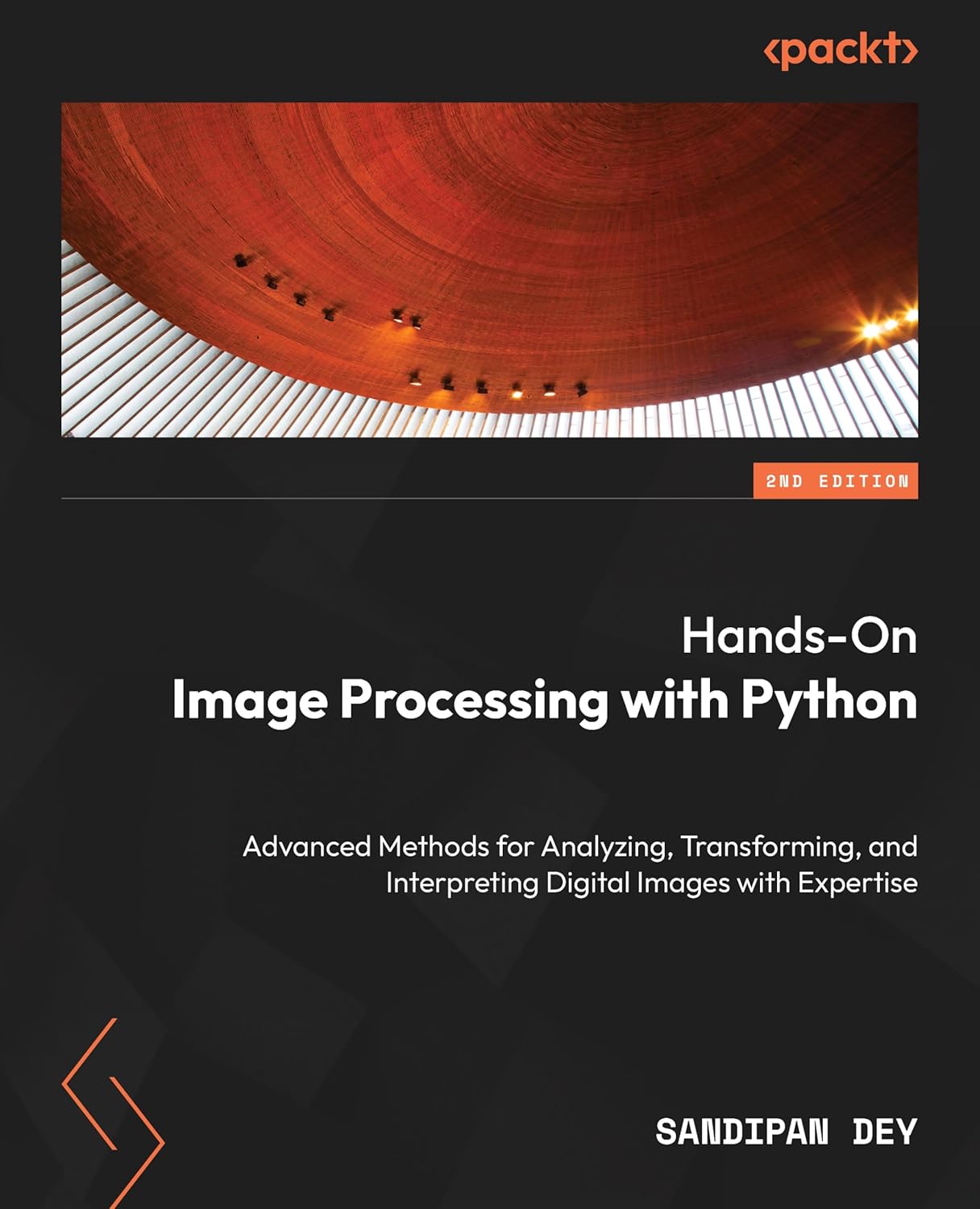
Table of Contents
Identifying and Extracting Scatological Data from Podcast Sources
Scatological data, while often dismissed, offers valuable insights into listener preferences, reactions, and engagement. This data can be found in various places: user comments on podcast hosting platforms, reviews on Apple Podcasts or Spotify, social media mentions (Twitter, Facebook, Instagram), and even feedback gathered through surveys or email interactions. Effectively harnessing this data requires efficient collection and preprocessing.
Automated Data Collection
AI significantly streamlines the collection of this data from disparate sources. Instead of manually sifting through countless comments and reviews, AI-powered tools can automate this process, saving you significant time and effort.
- Web scraping: Tools can automatically extract data from podcast websites and comment sections.
- API integrations: Direct access to platform APIs (like Spotify or Apple Podcasts) allows for automated data retrieval in structured formats.
- Social media monitoring tools: These tools track mentions of your podcast and relevant keywords on various social media platforms, capturing valuable user feedback.
- Sentiment analysis tools: These tools can automatically assess the emotional tone of user comments and reviews, helping to identify positive, negative, and neutral sentiment related to scatological content.
Data Cleaning and Preprocessing
Raw scatological data is often messy, containing irrelevant information, slang, profanity, and inconsistencies in language. Cleaning and preparing this data for analysis is crucial for accurate results.
- Natural Language Processing (NLP): NLP techniques are essential for parsing and understanding the unstructured text data found in comments and reviews.
- Stemming and Lemmatization: These processes reduce words to their root forms, improving the accuracy of analysis by grouping related words together (e.g., "shit," "shitty," and "shitting" are all related to the same core concept).
- Regular Expressions: Used to filter out irrelevant characters, symbols, and unwanted content from the data.
- Profanity filtering: While analyzing scatological data, tools can be used to identify and handle profanity appropriately, either by removing it or flagging it for separate analysis.
Analyzing Scatological Data with AI for Podcast Improvement
Once the data is cleaned and prepared, AI techniques can reveal valuable insights that can directly improve your podcast.
Sentiment Analysis and Topic Modeling
Sentiment analysis helps gauge listener reactions to episodes containing scatological humor or discussions. Positive sentiment indicates well-received content, while negative sentiment suggests areas for improvement.
- Identifying recurring themes: Sentiment analysis can identify common positive and negative themes within scatological discussions. For instance, if listeners consistently react negatively to a particular type of joke, you can adjust your future content.
- Emotional response analysis: Analyzing the emotional intensity of user reactions provides a deeper understanding of the impact of your scatological content on listeners.
- Topic modeling: This technique can group similar comments and reviews together, uncovering underlying topics and themes within the scatological data.
Trend Identification and Predictive Modeling
AI can analyze past data to identify emerging trends and predict future listener preferences related to scatological content.
- Predicting episode success: By analyzing past data, AI can predict the potential success of future episodes based on their scatological content.
- Identifying successful comedic styles: AI can identify trends in what types of scatological humor resonate most with your audience.
- Forecasting audience engagement: Using predictive modeling, you can forecast audience engagement based on the type and amount of scatological content included.
Transforming Insights into Actionable Podcast Strategies
The insights derived from analyzing scatological data should translate into tangible improvements in your podcast production and community management.
Content Strategy Optimization
Understanding listener reactions to scatological humor or discussions can directly inform your future episode planning.
- Refining episode topics: Based on the analysis, you can adjust your content strategy, focusing on topics that resonate positively with your audience and avoiding those that receive negative feedback.
- Guest selection: Data analysis can help in choosing guests who are likely to contribute to positive and engaging scatological discussions.
- Adjusting comedic style: Insights can guide you in refining your comedic approach to scatological humor, ensuring it aligns with your audience's preferences.
Community Engagement Enhancement
Identifying recurring concerns or preferences in scatological data enables more effective community management.
- Responding to listener feedback: By understanding the sentiment around specific scatological topics, you can craft better responses to listener comments and build stronger relationships.
- Building community guidelines: Data analysis can help refine your community guidelines and foster a more positive and inclusive environment for discussions involving scatological content.
- Proactive community management: By identifying potential concerns early, you can proactively address them, mitigating potential negative experiences for your audience.
Conclusion
AI-driven podcast creation is not just about technology; it's about understanding and responding to your audience. By effectively analyzing and transforming repetitive scatological data, you can unlock a wealth of insights that can significantly enhance your podcast’s success. Don't let this valuable data remain untapped. Embrace AI-driven tools and techniques to refine your content strategy, strengthen your community engagement, and ultimately, create a more impactful and successful podcast. Start leveraging the power of AI for analyzing and transforming your repetitive scatological data today!
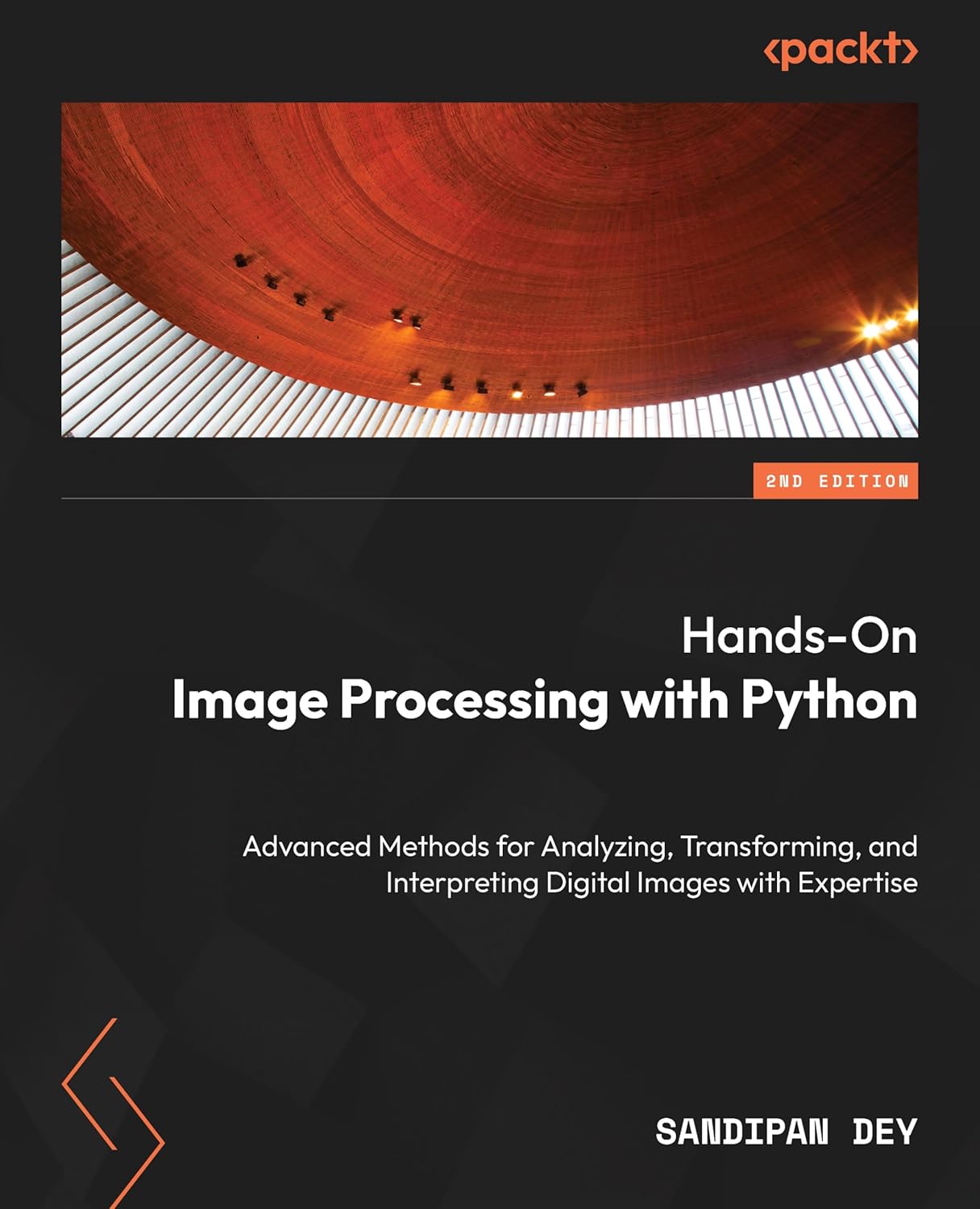
Featured Posts
-
Five Point Plan Unveiled By Canadian Auto Dealers Amidst Us Trade Tensions
Apr 24, 2025 -
China Diversifies Lpg Imports Middle East Replaces Us Amid Tariffs
Apr 24, 2025 -
This Startup Airline Is Profiting From Deportation Flights An Analysis
Apr 24, 2025 -
Potential Sale Of Utac Update On Chinese Buyout Firms Plans
Apr 24, 2025 -
Tesla Q1 2024 Lower Profits And The Musk Administration Connection
Apr 24, 2025
Latest Posts
-
Eu Must Do More To Counter Us Tariffs Says French Minister
May 10, 2025 -
France Demands Stronger Eu Response To Us Tariffs
May 10, 2025 -
Us Tariffs French Minister Pushes For Increased Eu Retaliation
May 10, 2025 -
Stronger Eu Response Needed To Us Tariffs French Ministers Statement
May 10, 2025 -
French Minister Calls For Stronger Eu Response To Us Tariffs
May 10, 2025