Understanding AI's "Thought" Processes: A Surprisingly Simple Explanation
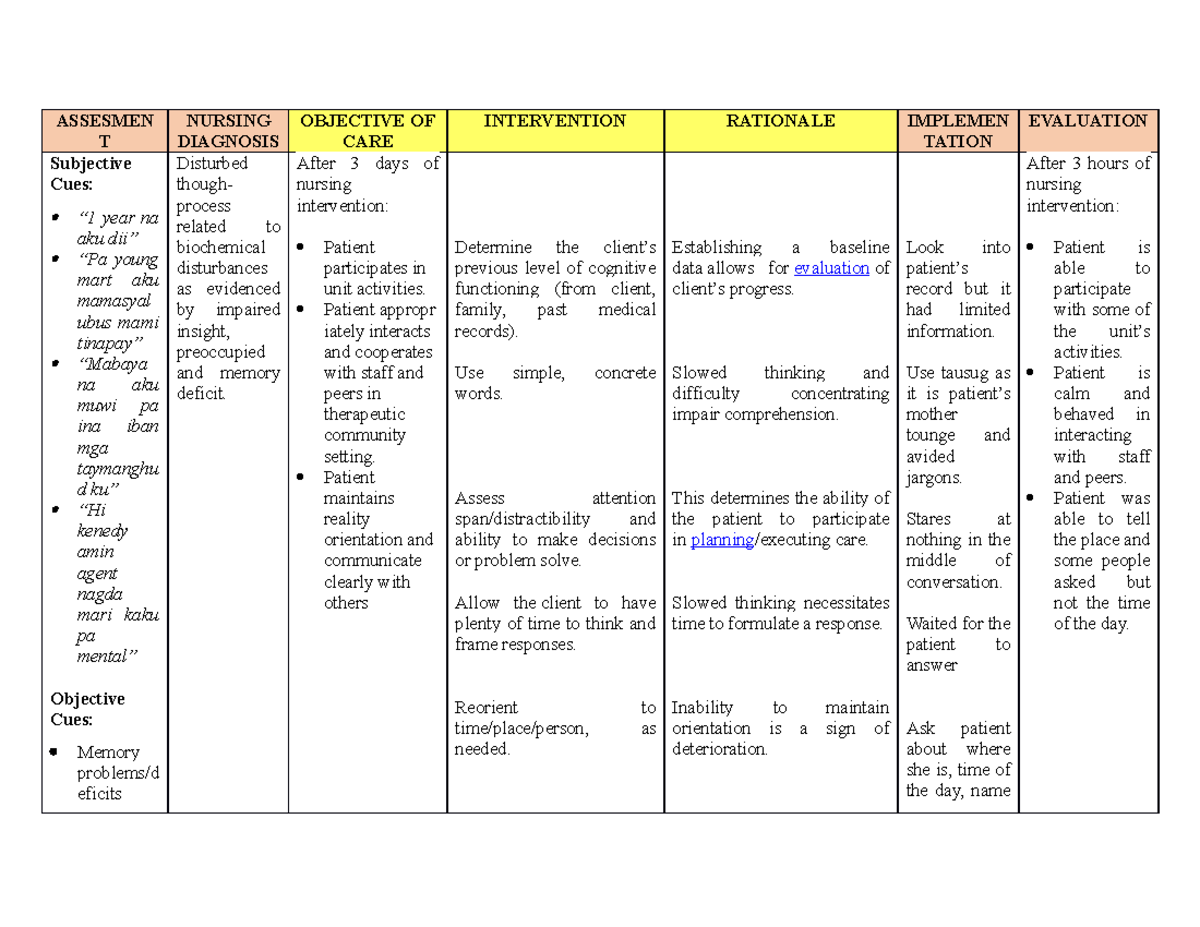
Table of Contents
AI Doesn't "Think" Like Humans: The Fundamental Difference
A crucial point to grasp is that AI, despite its capabilities, doesn't possess consciousness or subjective experience. It doesn't "think" in the same way humans do. Instead, AI operates based on algorithms and the data it's fed. Understanding this fundamental difference is key to comprehending AI's "thought" processes.
To illustrate, consider the difference between baking a cake using a recipe and creating a completely novel culinary masterpiece. The recipe provides a structured set of instructions—akin to an algorithm—that, when followed precisely, yields a predictable outcome. Human creativity, on the other hand, involves intuition, emotions, and a vast network of experiences that lead to unique and unpredictable results. AI, much like the cake recipe, follows predefined rules and established patterns.
- AI relies on statistical probability and pattern recognition. It identifies trends and makes predictions based on the data it has been trained on.
- Human thought involves emotions, intuition, and subjective experiences. These elements are currently absent in AI systems.
- AI decision-making is based on pre-programmed rules and training data. It doesn't possess independent reasoning or critical thinking in the human sense.
The Role of Algorithms in AI "Thinking"
Algorithms are the core of AI's "thought" processes. Simply put, an algorithm is a set of rules or instructions that dictates how AI processes information and arrives at a decision or prediction. Different types of algorithms underpin different AI capabilities.
- Algorithms are sets of rules that dictate how AI processes data. They determine the steps involved in analyzing information and generating an output.
- Machine learning algorithms learn from data without explicit programming. They identify patterns and adjust their internal parameters to improve their accuracy over time.
- Deep learning algorithms use artificial neural networks to analyze data in complex ways. These networks, inspired by the human brain, allow AI to handle vast and intricate datasets.
- Examples of algorithms used in different AI applications include: convolutional neural networks (CNNs) for image recognition, recurrent neural networks (RNNs) for natural language processing, and decision trees for classification tasks.
Data is the Fuel: How AI Learns and "Thinks"
Data is the lifeblood of AI. Without sufficient and relevant data, AI systems cannot learn or function effectively. The process of "training" an AI model involves feeding it massive amounts of data so it can identify patterns and relationships within that data. This data becomes the basis for its subsequent "thinking" and decision-making.
- Large datasets are crucial for training accurate and effective AI models. The more data an AI system processes, the better it typically performs.
- The quality and bias of training data significantly impact AI performance. Garbage in, garbage out – if the data is flawed, the AI's output will also be flawed.
- Data preprocessing and cleaning are essential steps in AI development. This involves removing inconsistencies, errors, and irrelevant information from the dataset.
- Ethical implications of biased datasets: Biased data can lead to AI systems perpetuating and even amplifying existing societal biases. This is a major concern in fields like criminal justice and loan applications.
Understanding Bias in AI: A Critical Perspective
Bias in AI is a significant concern. Because AI systems learn from data, they can inadvertently inherit and amplify existing biases present in that data. This can lead to unfair or discriminatory outcomes.
- AI systems reflect the biases present in their training data. If the data used to train an AI system is biased, the system itself will likely exhibit that bias.
- Addressing bias in AI requires careful data curation and algorithm design. This includes actively seeking diverse and representative datasets and designing algorithms that mitigate bias.
- The importance of diversity and inclusion in AI development teams: Diverse teams are better equipped to identify and address biases in data and algorithms.
Conclusion
Understanding AI's "thought" processes involves appreciating the fundamental differences between human cognition and algorithmic processing. AI doesn't "think" in the same way we do; rather, it relies on sophisticated algorithms and vast amounts of data to identify patterns and make predictions. While powerful, AI's reliance on data highlights the importance of addressing bias and ensuring ethical development.
Want to delve deeper into the fascinating world of AI? Explore more resources on AI algorithms and ethical considerations to gain a more comprehensive understanding of AI's "thought" processes and their implications for the future. Continue learning about how AI works and its impact on our lives – understanding AI's "thought" processes is crucial in navigating this rapidly evolving technological landscape.
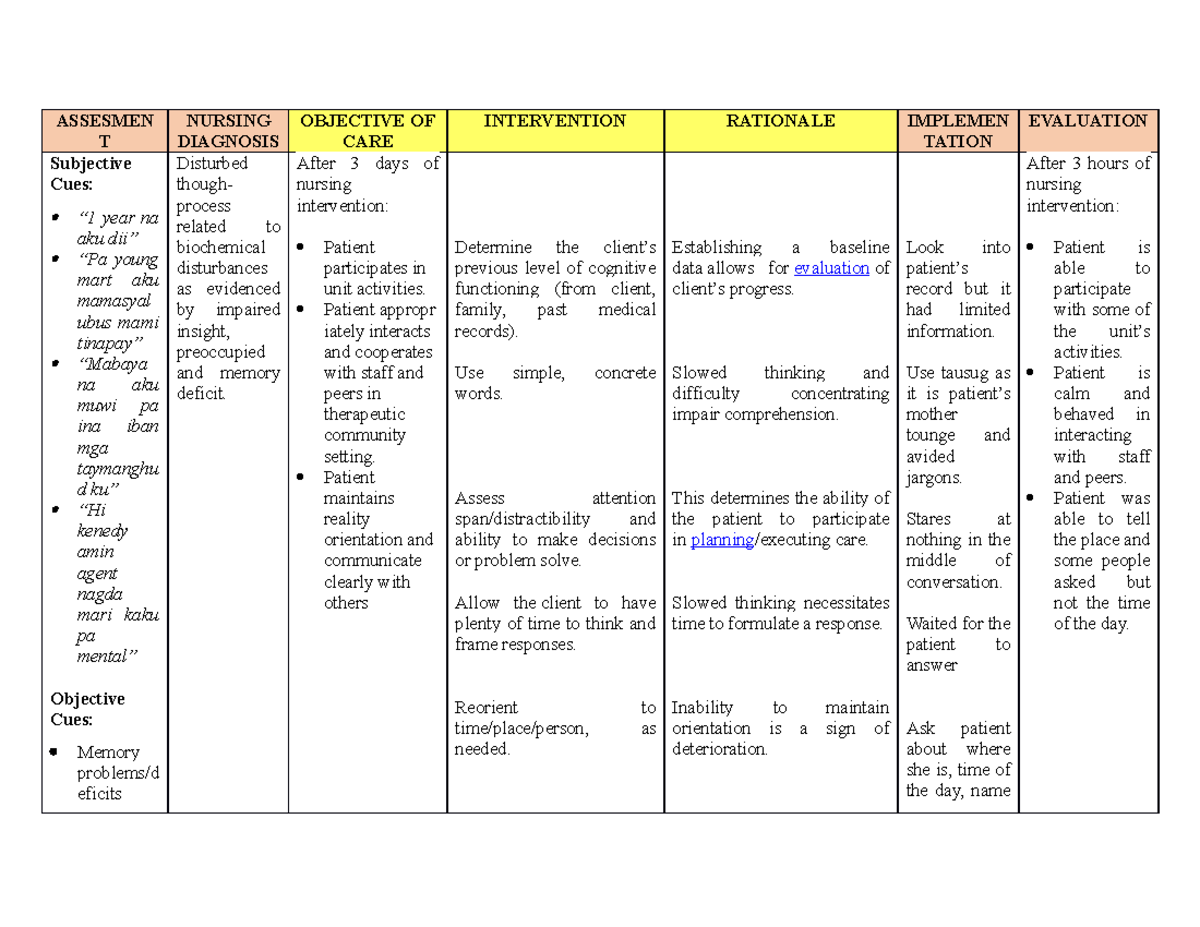
Featured Posts
-
India Fund Manager Dsp Sounds Warning Bell On Equities Boosts Cash Holdings
Apr 29, 2025 -
Analyzing The Effects Of Trumps Tariffs On Us Consumers And Businesses
Apr 29, 2025 -
Understanding The Russian Militarys Impact On European Security
Apr 29, 2025 -
Is Anthony Edwards Playing Tonight Timberwolves Vs Lakers Injury Report
Apr 29, 2025 -
Schumer Stays Put No Plans To Pass The Torch Says Senate Majority Leader
Apr 29, 2025
Latest Posts
-
Cybercriminal Accused Of Millions In Office365 Executive Email Theft
Apr 29, 2025 -
Federal Charges Filed Millions Stolen Through Executive Office365 Compromises
Apr 29, 2025 -
Office365 Data Breach Millions Stolen Suspect Arrested
Apr 29, 2025 -
Ohio Train Derailment Aftermath Prolonged Presence Of Toxic Chemicals In Buildings
Apr 29, 2025 -
Months Long Lingering Of Toxic Chemicals From Ohio Train Derailment In Buildings
Apr 29, 2025