Legacy Systems And AI: A Recipe For Disaster?
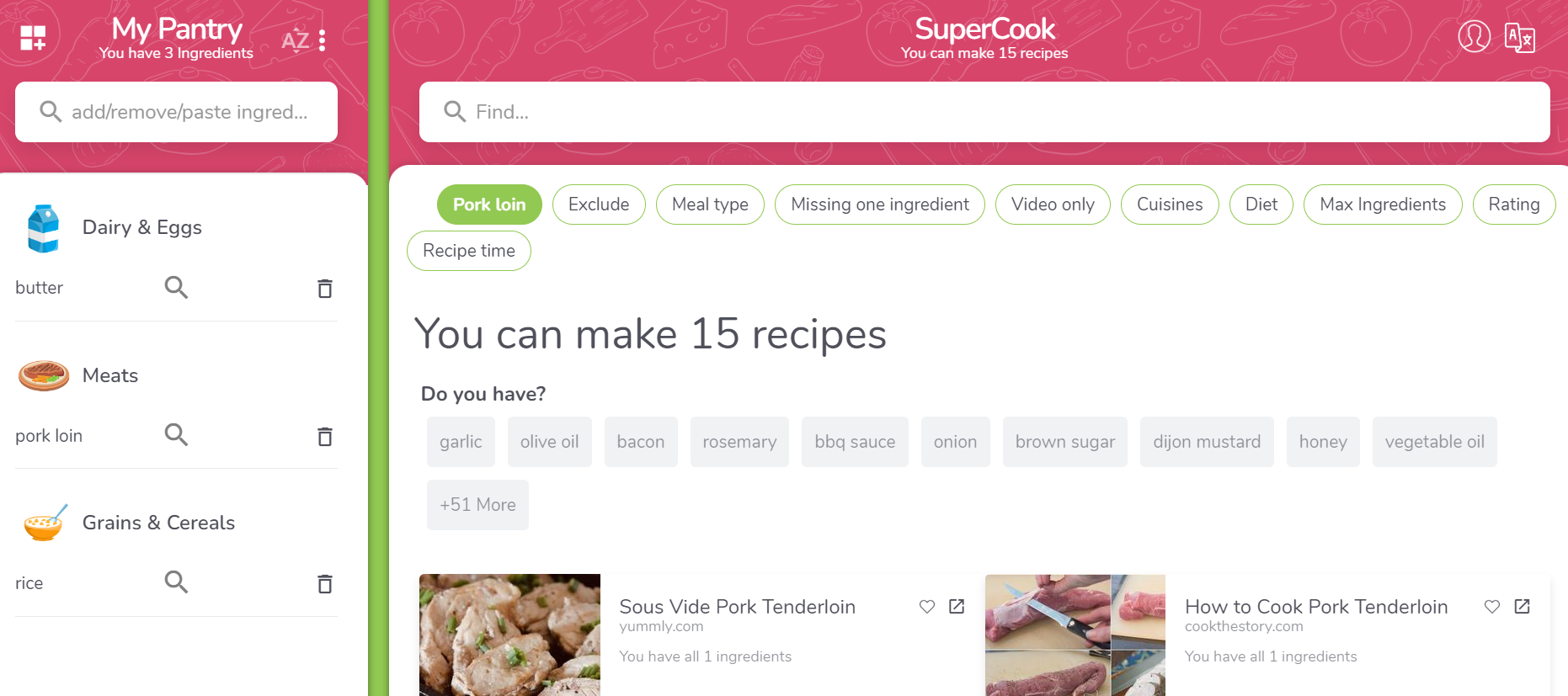
Table of Contents
Legacy systems, typically defined as outdated information systems built on older technologies, often suffer from several key characteristics: inflexible architecture, poor documentation, limited scalability, and a lack of modern security features. The integration of AI, with its demands for high-quality data and modern infrastructure, presents a significant clash with these limitations. The potential for conflict between modern AI and legacy systems is substantial, creating a need for careful planning and execution. This article aims to highlight these challenges and provide practical strategies to ensure a smooth and secure integration of legacy systems and AI.
The Challenges of Integrating AI with Legacy Systems
The integration of AI with legacy systems is far from a seamless process. Several significant hurdles must be overcome to ensure a successful outcome.
Data Incompatibility
One of the most significant obstacles is data incompatibility. AI models thrive on high-quality, clean, and readily accessible data. Legacy systems, however, often store data in disparate formats, creating significant challenges.
- Different data formats: Legacy systems frequently use outdated data formats incompatible with modern AI tools.
- Lack of data standardization: Inconsistent data structures and naming conventions hinder data integration and analysis.
- Data silos: Data may be scattered across multiple, unconnected systems, making it difficult to build a comprehensive dataset for AI training.
- Data quality issues: Inaccurate, incomplete, or outdated data within legacy systems can significantly impact the accuracy and reliability of AI models.
These data challenges directly affect the performance of AI models, potentially leading to inaccurate predictions, flawed decision-making, and ultimately, project failure. Thorough data cleansing and transformation are crucial for successful legacy systems and AI integration.
Integration Complexity
Connecting AI systems to legacy architectures presents significant technical hurdles.
- Lack of APIs: Many legacy systems lack the Application Programming Interfaces (APIs) necessary for seamless data exchange with modern AI systems.
- Outdated programming languages: Integrating with systems built using outdated languages like COBOL or Fortran requires specialized skills and expertise, increasing development time and costs.
- Security concerns: Integrating AI systems with legacy systems often exposes vulnerabilities in older security protocols.
- Limited scalability: Legacy systems may struggle to handle the increased data volume and processing demands of AI applications.
The cost and time associated with overcoming these integration complexities can be substantial, requiring significant investment in both personnel and technology.
Security Risks
The integration of AI with legacy systems also introduces heightened security risks.
- Weaknesses in legacy security protocols: Older systems often lack the robust security features of modern applications, creating vulnerabilities that malicious actors can exploit.
- Potential data breaches: Integrating AI may expose sensitive data stored within legacy systems to potential breaches, leading to significant financial and reputational damage.
- Lack of robust access controls: Insufficient access control mechanisms can allow unauthorized access to sensitive information used by AI systems.
Implementing robust security measures throughout the integration process is paramount to mitigate these risks. This includes regular security audits, penetration testing, and the implementation of strong access control mechanisms.
Mitigating the Risks: Strategies for Successful Integration
Successfully integrating AI with legacy systems requires a strategic and phased approach.
Modernization Strategies
Modernizing legacy systems is often necessary to facilitate AI integration. Several strategies exist:
- Refactoring: Restructuring the existing codebase to improve its design and maintainability.
- Rehosting: Moving the legacy system to a cloud environment without significant code changes.
- Replatforming: Migrating the system to a new platform while retaining the core functionality.
- Replacing: Replacing the legacy system entirely with a modern alternative.
- Retiring: Decommissioning the legacy system if it's no longer essential.
Each strategy has pros and cons regarding cost, time, and complexity. Careful evaluation is essential to select the most appropriate approach.
Data Wrangling and Transformation
Effective data preparation is crucial for successful AI integration.
- Data cleaning: Identifying and correcting inaccuracies, inconsistencies, and missing values in the data.
- Data transformation: Converting data into a format suitable for AI algorithms.
- ETL processes: Using Extract, Transform, Load (ETL) tools to automate data extraction, transformation, and loading into AI systems.
- Data enrichment: Augmenting existing data with external sources to enhance its quality and completeness.
The quality of data directly impacts the accuracy and reliability of AI models. Investing in robust data preparation techniques is essential.
Incremental Approach
Adopt a phased approach, starting with small, less critical applications.
- Pilot projects: Start with small-scale pilot projects to test the feasibility of AI integration.
- Proof-of-concept: Develop a proof-of-concept to demonstrate the potential benefits of AI integration.
- Iterative development: Use an iterative approach, allowing for learning and adaptation based on early results.
This minimizes risks and allows for adjustments based on experience.
Robust Security Measures
Implement comprehensive security protocols throughout the integration process.
- Regular security audits: Conduct regular audits to identify and address potential security vulnerabilities.
- Penetration testing: Simulate cyberattacks to identify weaknesses in the system.
- Access control management: Implement robust access control mechanisms to restrict access to sensitive data.
- Data encryption: Encrypt sensitive data both in transit and at rest to protect it from unauthorized access.
Proactive security measures are crucial to prevent breaches and maintain data integrity.
Conclusion: Avoiding a Legacy Systems and AI Disaster
Integrating AI with legacy systems presents significant challenges, including data incompatibility, integration complexity, and increased security risks. However, by adopting a strategic approach that includes modernization strategies, robust data preparation techniques, an incremental implementation plan, and comprehensive security measures, organizations can successfully harness the power of AI while mitigating potential risks. Successful integration offers substantial rewards: improved efficiency, enhanced decision-making, and the potential for new revenue streams. Don't let legacy systems and AI become a recipe for disaster. Plan your integration carefully, and contact us to explore modernization solutions for your legacy systems and AI integration needs.
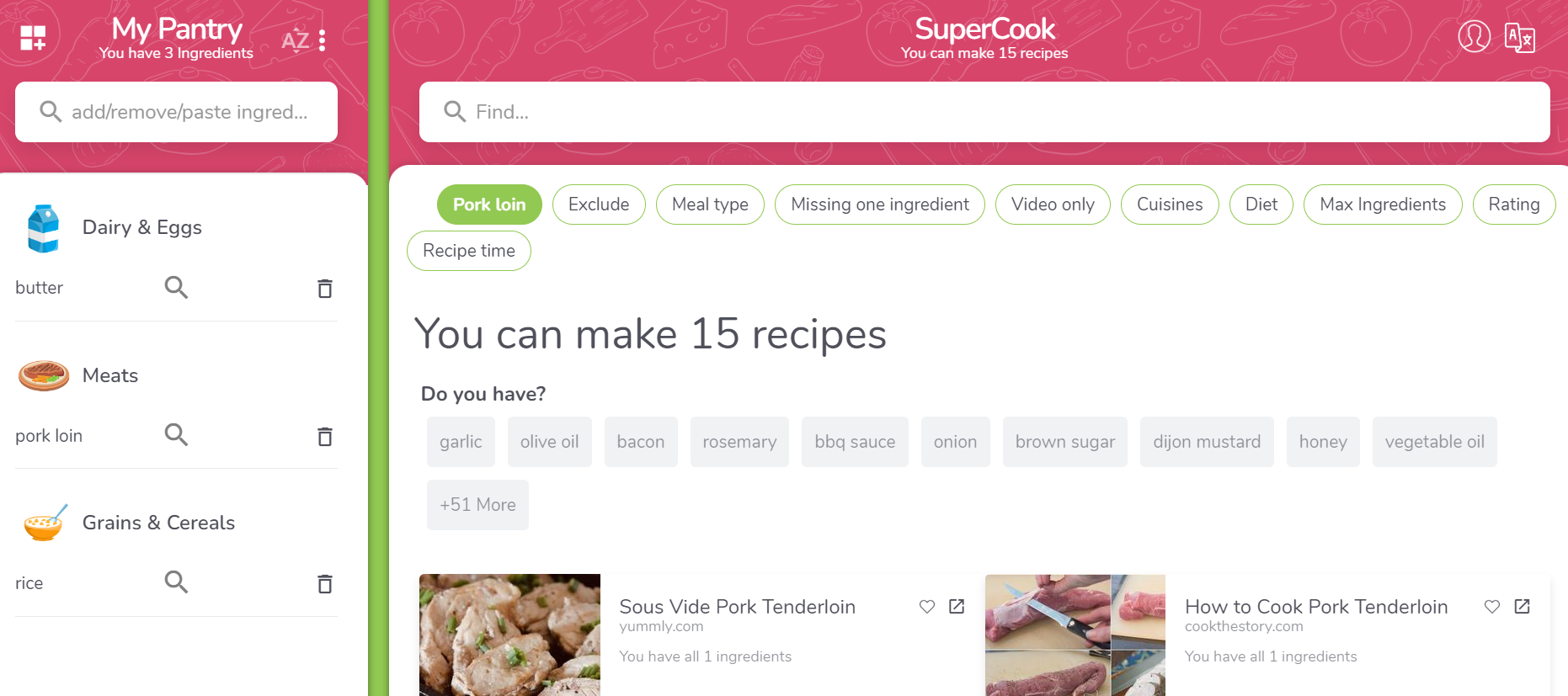
Featured Posts
-
Impact Of Warmer Weather On Russias Military Operations In Ukraine
Apr 30, 2025 -
Resilient Investments Power China Lifes Profit Growth
Apr 30, 2025 -
Disney Cruise Line Expands Alaska Offerings For Summer 2026
Apr 30, 2025 -
Remember Mondays Eurovision Journey Fighting Online Hate Through Song
Apr 30, 2025 -
Your Daily Horoscope Astrological Predictions For April 17 2025
Apr 30, 2025