Legacy Business Applications And The AI Challenge
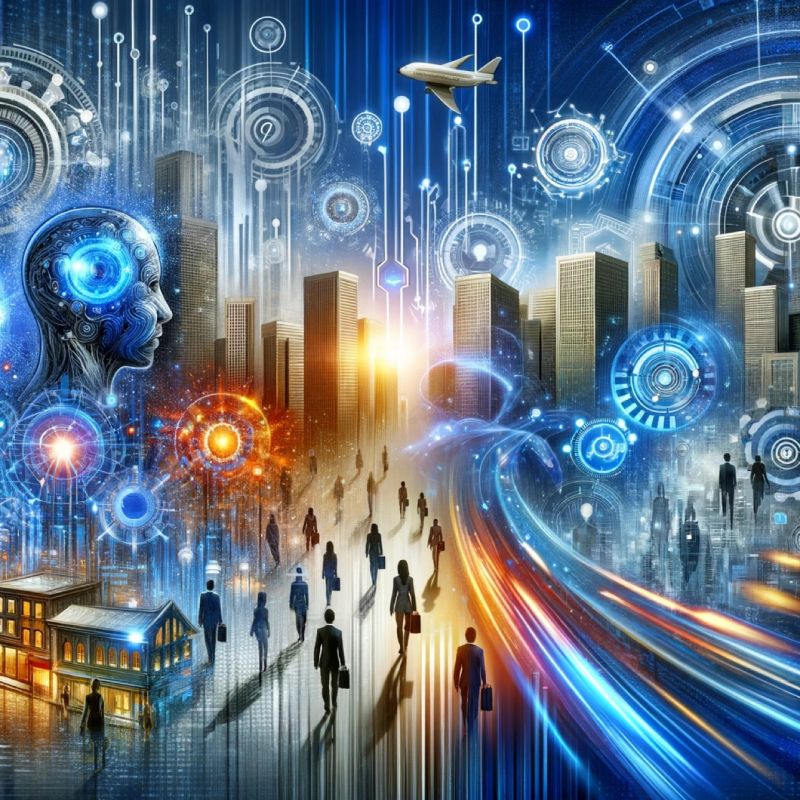
Table of Contents
The Challenges of Integrating AI with Legacy Systems
Integrating AI into an environment dominated by legacy applications presents a multi-faceted challenge. The complexities stem from several key areas:
Data Accessibility and Compatibility Issues
Legacy systems often suffer from:
- Data Silos: Data is scattered across various, unconnected systems, hindering comprehensive analysis.
- Incompatible Data Formats: Inconsistent data structures and formats make it difficult to consolidate and process information effectively.
- Lack of APIs: The absence of Application Programming Interfaces (APIs) makes it hard to integrate legacy systems with modern AI tools and platforms.
- Difficulty Extracting Relevant Data: Locating and extracting the specific data required for AI model training can be a time-consuming and complex process.
These issues directly impact AI model training, often resulting in:
- Difficulty training accurate and reliable AI models due to incomplete or inconsistent data.
- Inaccurate predictions and flawed decision-making based on incomplete or biased data.
- Significant limitations in the overall capabilities of the implemented AI solutions.
Infrastructure Limitations and Scalability
Legacy systems are frequently hampered by:
- Outdated Hardware: Old servers and infrastructure often lack the processing power required for demanding AI workloads.
- Lack of Cloud Infrastructure: Many legacy systems are not designed for cloud deployment, limiting scalability and flexibility.
- Insufficient Processing Power for AI Workloads: AI algorithms require substantial computational resources, which may be lacking in legacy system environments.
- Security Concerns: Older systems may have vulnerabilities that pose security risks when integrating with AI, requiring significant investment in upgrades.
These limitations lead to:
- Performance bottlenecks and slow processing speeds, hindering AI functionality.
- High implementation costs associated with upgrading hardware and infrastructure.
- Increased vulnerability to security breaches and data loss.
Integration Complexity and Cost
Successfully integrating AI with legacy systems is a complex undertaking, involving:
- High Cost of Migration: Migrating data and functionalities from legacy systems to modern platforms can be expensive.
- Complexity of Integrating AI with Existing Systems: Connecting disparate systems and ensuring seamless data flow requires specialized expertise.
- Potential for Disruption to Existing Workflows: The integration process can temporarily disrupt business operations, causing delays and inefficiencies.
The implications of these complexities include:
- Significant project delays as integration proves more complex than anticipated.
- Budget overruns due to unforeseen costs and extended timelines.
- A higher risk of project failure due to the inherent complexities.
Strategies for Overcoming the Challenges
While the challenges are significant, several strategies can pave the way for successful AI integration with legacy systems:
Modernization Strategies: Gradual vs. Complete Overhaul
Businesses need to carefully consider their modernization approach:
- Refactoring: Rewriting parts of the legacy system to improve its structure and functionality.
- Rehosting: Migrating the legacy system to a cloud environment without significant code changes.
- Replacing: Replacing the legacy system entirely with a modern, cloud-based application.
- Phased Implementation: A gradual approach focusing on integrating AI with specific modules or functionalities before a complete overhaul.
The optimal approach depends heavily on individual circumstances, including budget constraints, business needs, and the complexity of the existing system. A phased approach is often the most practical and cost-effective starting point.
Data Integration and Cleansing Techniques
Effective data management is crucial:
- ETL (Extract, Transform, Load) Processes: Automated processes to extract data from various sources, transform it into a consistent format, and load it into a data warehouse.
- Data Warehousing: Creating a centralized repository for all relevant data, facilitating efficient access and analysis.
- Data Quality Management: Implementing processes to ensure data accuracy, completeness, and consistency.
- API Development: Creating APIs to enable seamless data exchange between legacy systems and modern AI tools.
Improved data management ensures the quality of data feeding AI models, leading to more accurate and reliable results.
Leveraging AI for Legacy System Enhancement
AI can even enhance existing legacy systems:
- Legacy System Monitoring: AI can detect anomalies and potential issues in real-time, enabling proactive maintenance.
- Automated Testing: AI-powered testing tools can automate regression testing, improving software quality and reducing manual effort.
- Predictive Maintenance: AI can analyze system performance data to predict potential failures, allowing for proactive maintenance and preventing downtime.
These applications of AI can lead to significant cost savings and efficiency gains by optimizing resource utilization and minimizing downtime.
Real-World Examples and Case Studies
Several companies have successfully integrated AI with their legacy systems. For instance, a major financial institution used AI to automate fraud detection within its core banking system, significantly reducing losses. A large manufacturing company implemented AI-powered predictive maintenance on its legacy machinery, resulting in reduced downtime and improved operational efficiency. These case studies, across diverse industries, showcase the tangible benefits of strategic AI integration with legacy systems.
Conclusion: Navigating the Future of Legacy Business Applications and AI
Integrating AI with legacy business applications presents significant challenges related to data accessibility, infrastructure limitations, and integration complexity. However, strategic modernization, improved data management, and the innovative application of AI for legacy system enhancement can overcome these hurdles. By adopting a phased approach and focusing on data quality, businesses can unlock the potential of AI to improve efficiency, reduce costs, and gain a significant competitive advantage. Don't let legacy business applications hold back your AI transformation – start planning your modernization strategy today!
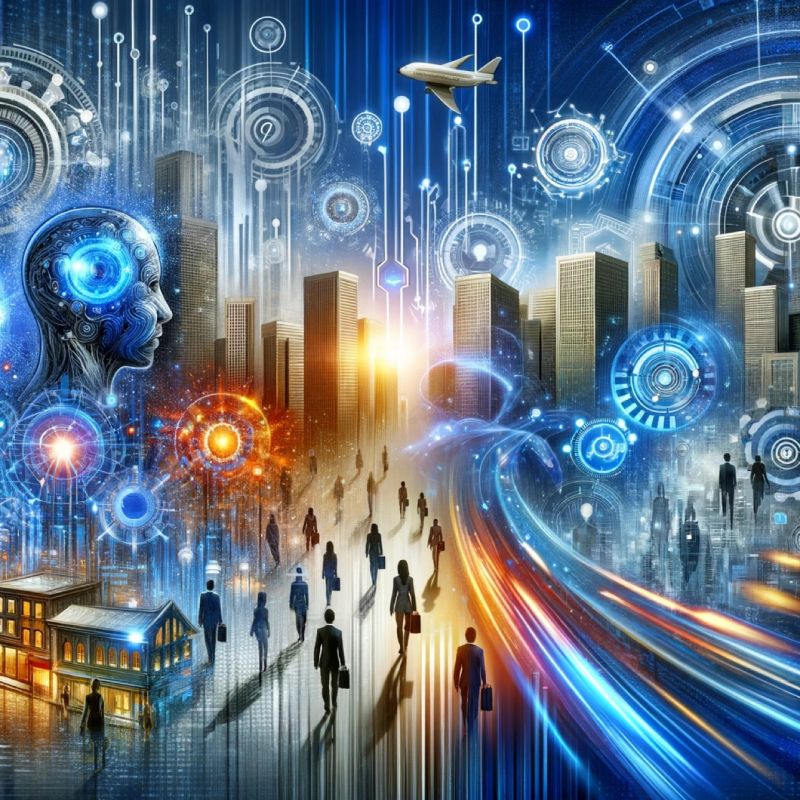
Featured Posts
-
A Reflective Prince William Kensington Palaces New Image
May 01, 2025 -
Six Nations Frances Convincing Victory Under Ramoss Leadership
May 01, 2025 -
Agha Syd Rwh Allh Mhdy Bharty Hkwmt Ky Kshmyr Palysy Ky Shdyd Mdhmt
May 01, 2025 -
4 14
May 01, 2025 -
The Impact Of The Target Boycott Analyzing The Response To Reduced Dei Efforts
May 01, 2025
Latest Posts
-
Independent Office For Police Conduct Iopc Challenges Bbc Panorama Over Chris Kaba
May 01, 2025 -
Panoramas Chris Kaba Episode Iopc Complaint Filed With Ofcom
May 01, 2025 -
Chris Kaba Police Watchdogs Formal Ofcom Complaint Explained
May 01, 2025 -
Police Watchdog Targets Panoramas Chris Kaba Coverage Ofcom Complaint Details
May 01, 2025 -
Chris Kaba Panorama Police Watchdog Complaint To Ofcom
May 01, 2025