Using AI To Create Engaging Podcasts From Repetitive Scatological Data
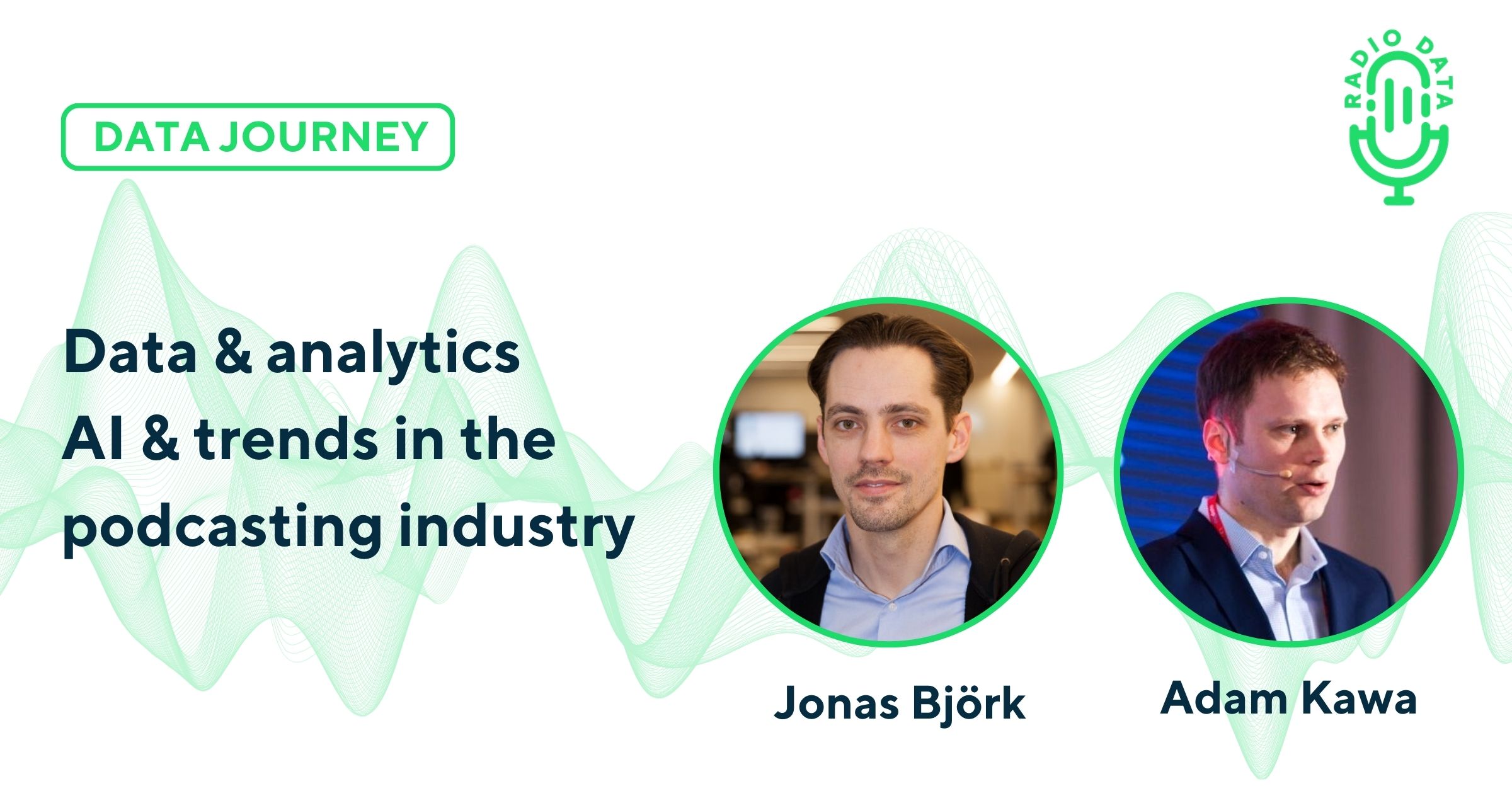
Table of Contents
Data Preprocessing and Cleaning for AI Podcast Generation
Before AI can weave its magic, the scatological data needs careful preparation. This stage, crucial for successful AI podcast generation, involves rigorous cleaning and transformation to make the data AI-ready.
Identifying and Handling Noise
Raw scatological data is often noisy, containing irrelevant information that can confuse the AI model. Effective scatological data cleaning is essential. This involves:
- Outlier Removal: Identifying and removing data points that deviate significantly from the norm.
- Smoothing: Applying techniques to reduce the impact of random fluctuations and noise in the data.
- Handling Missing Data: Implementing strategies to address missing values, such as imputation or removal, depending on the nature and extent of the missing data.
- Dealing with Inconsistencies: Standardizing data formats and resolving inconsistencies to ensure data integrity. This might involve correcting errors in data entry or applying consistent units of measurement.
This AI data preprocessing step is critical for improving the accuracy and reliability of the AI model's output. Effective noise reduction for audio in the later stages also relies heavily on clean, well-organized source data.
Data Transformation and Feature Engineering
Once cleaned, the raw scatological data needs transformation into a format suitable for AI models. This is where data transformation for AI becomes key. This involves:
- Data Normalization: Scaling the data to a standard range, preventing features with larger values from dominating the model.
- Feature Scaling: Adjusting the range of features to ensure they contribute equally to the AI model.
- Creating Relevant Features: Engineering new features from the existing data that better capture the essence of the information for narrative generation. This might involve calculating aggregate statistics or creating time-series representations.
This stage of feature engineering for podcasts directly impacts the quality and relevance of the AI-generated narrative. The goal is to create AI-ready scatological data that the AI model can effectively understand and utilize.
Selecting the Right AI Model for Podcast Creation
Choosing the appropriate AI model is paramount for generating a high-quality podcast. The right model will depend on the nature and structure of your data.
Natural Language Processing (NLP) Techniques
NLP for podcast generation is the heart of this process. Several powerful NLP models can be employed, each with its strengths and weaknesses:
- GPT-3 (and its successors): Known for its ability to generate human-quality text, GPT-3 can create engaging narratives from structured or semi-structured data.
- BERT: Excellent for understanding context and relationships within the data, BERT can be used to create more nuanced and coherent narratives.
The choice between these and other models depends on factors like data size, desired narrative style, and computational resources. Effectively using scatological data NLP requires careful consideration of these factors. The goal is to achieve seamless AI narrative generation.
Text-to-Speech (TTS) and Audio Enhancement
Once the AI generates a script, it needs to be converted into an audio podcast. This requires:
- Choosing Appropriate TTS Engines: Selecting a TTS engine that produces natural-sounding speech, capable of conveying the tone and style desired for the podcast.
- Audio Editing and Mastering: Refining the audio output, adding music, sound effects, and performing other edits to achieve a professional sound. This involves podcast audio mastering techniques to enhance clarity and listener engagement.
Careful selection of TTS engines and diligent audio editing contribute to high-quality audio enhancement for scatological data podcasts, transforming the AI-generated text into a polished and professional-sounding podcast. The use of AI-powered TTS is increasingly sophisticated, resulting in more human-like audio output.
Ensuring Ethical Considerations and Data Privacy
Handling sensitive scatological data requires stringent adherence to ethical guidelines and data protection regulations.
Anonymization and Data Security
Protecting individual privacy is paramount. This requires:
- Data Anonymization Techniques: Employing methods to remove or alter personally identifiable information from the data before processing.
- Secure Data Storage and Handling Procedures: Implementing robust security measures to protect the data throughout the entire process, complying with regulations like GDPR.
Data privacy for podcasts using sensitive information is crucial. Implementing secure secure AI processing is non-negotiable.
Responsible AI and Bias Mitigation
AI models can inherit biases present in the training data. To create a responsible and ethical podcast:
- Bias Detection and Mitigation Techniques: Employing methods to identify and mitigate potential biases in the AI model's output.
- Ensuring Fairness and Inclusivity: Taking steps to ensure the generated content is fair, unbiased, and inclusive.
This focus on responsible AI and bias mitigation in NLP is critical for creating ethical AI podcast creation.
Conclusion
Using AI to create engaging podcasts from repetitive scatological data offers significant advantages. This process involves careful data preprocessing and cleaning, selecting the appropriate AI model for narrative generation and text-to-speech conversion, and meticulous attention to ethical considerations and data privacy. The key takeaways are increased efficiency in content creation, the ability to overcome limitations of traditional podcasting methods when dealing with complex data, and the creation of engaging content from seemingly unworkable datasets.
Leverage AI to transform your scatological data into compelling podcasts. Explore the power of AI in creating engaging podcasts from even the most challenging datasets. For further reading on AI-powered podcast creation and data privacy best practices, explore resources from leading AI research institutions and data privacy organizations.
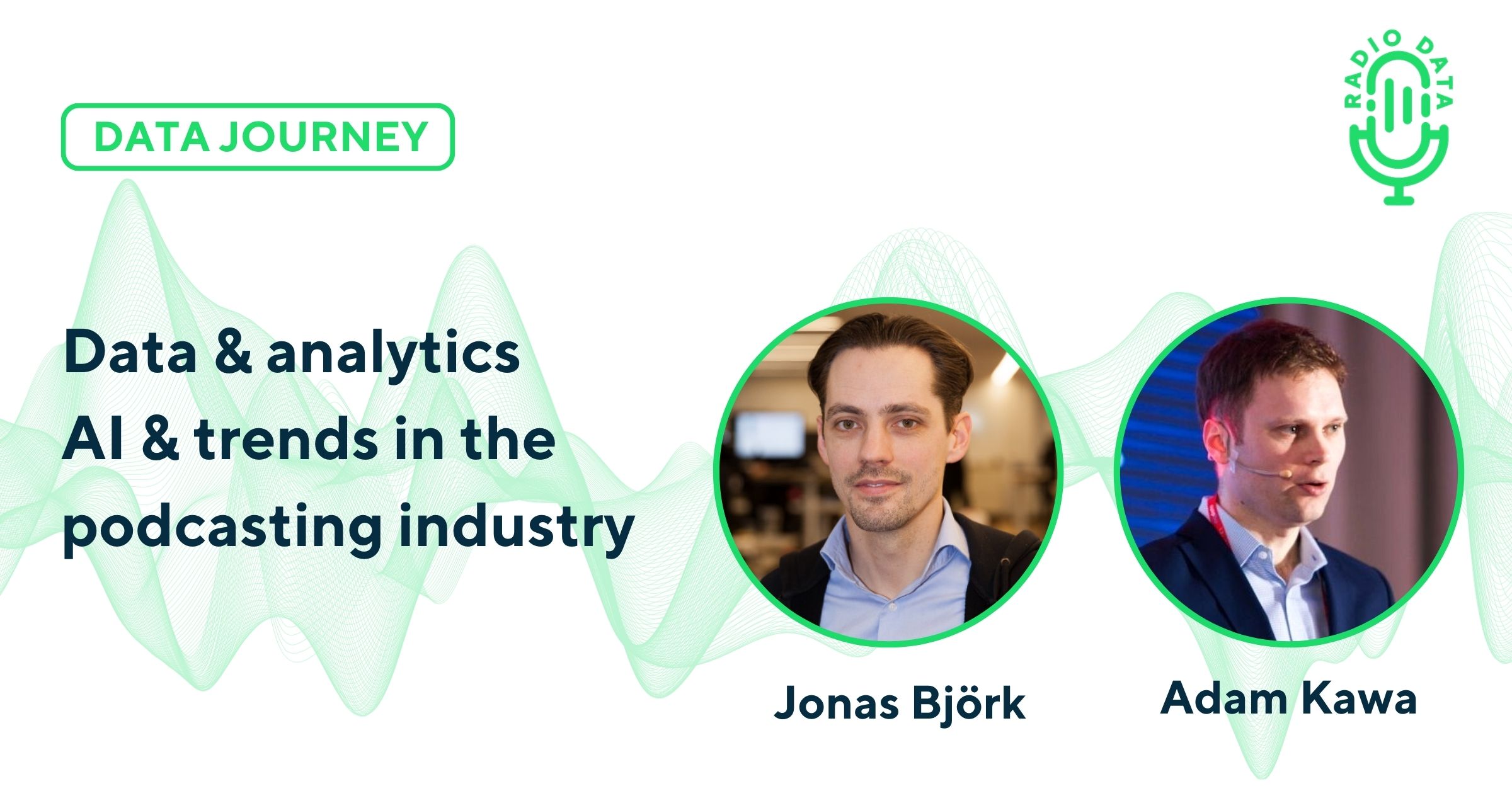
Featured Posts
-
Mariners First Inning Explosion Leads To 14 0 Victory Against Marlins
May 07, 2025 -
Steelers Pickens A Potential 2026 Departure
May 07, 2025 -
Middle Management Their Value To Companies And Their Teams
May 07, 2025 -
Ripples Xrp Soars Can It Sustain Momentum And Hit 3 40
May 07, 2025 -
The Most Hilarious April Fools Pranks In History
May 07, 2025
Latest Posts
-
The White Lotus Season 3 Unmasking The Voice Of Kenny
May 07, 2025 -
White Lotus Latest Episode Features Surprise Oscar Winner
May 07, 2025 -
Oscar Winner Makes White Lotus Appearance A Deeper Look
May 07, 2025 -
Ke Huy Quans The White Lotus Cameo A Hidden Gem For Fans
May 07, 2025 -
The White Lotus Season 2 Ke Huy Quans Unexpected Voice Appearance
May 07, 2025