Successfully Implementing The CNIL's New AI Model Guidelines
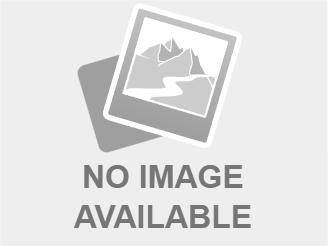
Table of Contents
Understanding the Core Principles of the CNIL AI Guidelines
The CNIL's AI guidelines reinforce the principles enshrined in the GDPR (General Data Protection Regulation), focusing on how these apply specifically to AI systems. Understanding these core principles is foundational for successful implementation. Key principles include:
-
Data Protection by Design and by Default: Privacy must be integrated into the design and development process from the outset, not as an afterthought. This includes minimizing data collection and implementing robust security measures. This aligns with Article 25 of the GDPR.
-
Purpose Limitation: AI systems should only process personal data for specified, explicit, and legitimate purposes. The purpose should be clearly defined and adhered to throughout the AI's lifecycle. This is directly related to Article 5(1)(b) of the GDPR.
-
Data Minimization: Only collect and process the data strictly necessary for the AI's purpose. Avoid unnecessary data collection. This principle, crucial for minimizing risk, is echoed in Article 5(1)(c) of the GDPR.
-
Accuracy: Ensure the data processed by the AI system is accurate and kept up to date. Regular data quality checks are vital for compliance.
-
Transparency: Users should be informed about the use of AI in decision-making processes that affect them. This includes understanding the logic involved and having access to information about the data used. This relates to Article 13 and 14 of the GDPR.
-
Accountability: Organizations must be able to demonstrate compliance with the CNIL's guidelines. This includes maintaining detailed records of data processing activities and implementing appropriate security measures. This directly addresses Article 5(2) of the GDPR.
Data Governance and Risk Assessment under the CNIL Framework
Thorough data governance is paramount for complying with the CNIL's AI framework. This involves establishing clear roles and responsibilities, implementing robust data security measures, and conducting regular data protection impact assessments (DPIAs).
-
Conducting a DPIA: A comprehensive DPIA for AI systems should specifically address risks related to:
- Bias and Discrimination: Identify and mitigate potential biases in data and algorithms that could lead to discriminatory outcomes.
- Security Breaches: Assess the risks of unauthorized access, use, disclosure, alteration, or destruction of personal data processed by the AI system.
- Data Integrity: Evaluate the risks of inaccurate, incomplete, or outdated data impacting the AI's performance and decisions.
-
Identifying and Mitigating Risks: Implement appropriate technical and organizational measures to mitigate identified risks. This might include data anonymization techniques, access control mechanisms, and regular security audits.
-
CNIL Expectations on Data Security: The CNIL expects robust security measures, including encryption, access controls, and regular vulnerability assessments, to protect personal data processed by AI systems. Failure to meet these expectations can result in significant penalties.
Ensuring Transparency and Explainability in AI Systems
Transparency and explainability are critical aspects of the CNIL's AI guidelines. Organizations must ensure users understand how AI systems are used and the impact on their lives.
-
Methods for Ensuring Transparency:
- Provide clear and accessible information about how the AI system works and the data it uses.
- Offer individuals the ability to challenge AI-driven decisions that affect them.
- Implement mechanisms for users to obtain human review of AI decisions.
-
Techniques for Explainable AI (XAI): Employ techniques that make the AI's decision-making process more understandable, such as providing explanations for individual decisions or visualizing the AI's internal workings.
-
Right to Explanation: Individuals have a right to a meaningful explanation of AI-driven decisions that significantly affect their rights and freedoms.
Practical Steps for Implementation and Ongoing Compliance
Implementing the CNIL's guidelines requires a structured approach:
- Create an internal policy: Develop a comprehensive policy outlining procedures for complying with the CNIL AI guidelines.
- Train employees: Educate staff on data protection principles, AI ethics, and the specific requirements of the CNIL guidelines.
- Implement technical safeguards: Put in place robust technical measures to secure personal data processed by AI systems.
- Conduct regular audits: Perform regular audits to assess compliance with the guidelines and identify areas for improvement.
- Establish a complaint handling mechanism: Set up a clear process for handling complaints related to the use of AI systems.
- Integrate with existing policies: Integrate the CNIL guidelines into your broader data protection policies and procedures.
- Ongoing monitoring and adaptation: Continuously monitor the regulatory landscape and adapt your practices to keep pace with evolving requirements.
Leveraging Technology for Compliance with CNIL AI Guidelines
Technology can significantly aid in compliance:
- Data Governance Platforms: These tools help manage data lifecycles, access control, and data quality, streamlining compliance efforts.
- AI Risk Assessment Tools: Automated tools can help identify and assess potential risks associated with AI systems, improving the efficiency of DPIAs.
- Privacy-Enhancing Technologies (PETs): Techniques like differential privacy and federated learning allow for data analysis while preserving individual privacy.
- AI Auditing Tools: These tools can monitor AI systems for bias, discrimination, and security vulnerabilities.
Conclusion
Successfully implementing the CNIL's AI model guidelines requires a proactive and comprehensive approach. By understanding the core principles, conducting thorough risk assessments, ensuring transparency, and leveraging technology, organizations can effectively navigate these regulations and foster trust. Don't let non-compliance hinder your AI projects. Start implementing the CNIL AI guidelines today to ensure a future of ethical and responsible AI development and deployment. Take the first step towards compliance by [link to relevant resource/contact information].
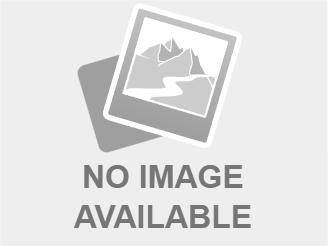
Featured Posts
-
Palmeiras Jogador Vomita Na Altitude E Deixa O Jogo
Apr 30, 2025 -
Soap Star Reveals Truth Behind Weepy Coronation Street Farewell
Apr 30, 2025 -
Kamala Harris Leadership Questioned After Election Silence
Apr 30, 2025 -
The Best New Cruises Setting Sail From The South In 2025
Apr 30, 2025 -
Kontsertnye Nomera Superboula 2025 Dzhey Zi Teylor Svift I Drugie
Apr 30, 2025