Overcoming Hurdles In Automated Visual Inspection Of Lyophilized Drug Products
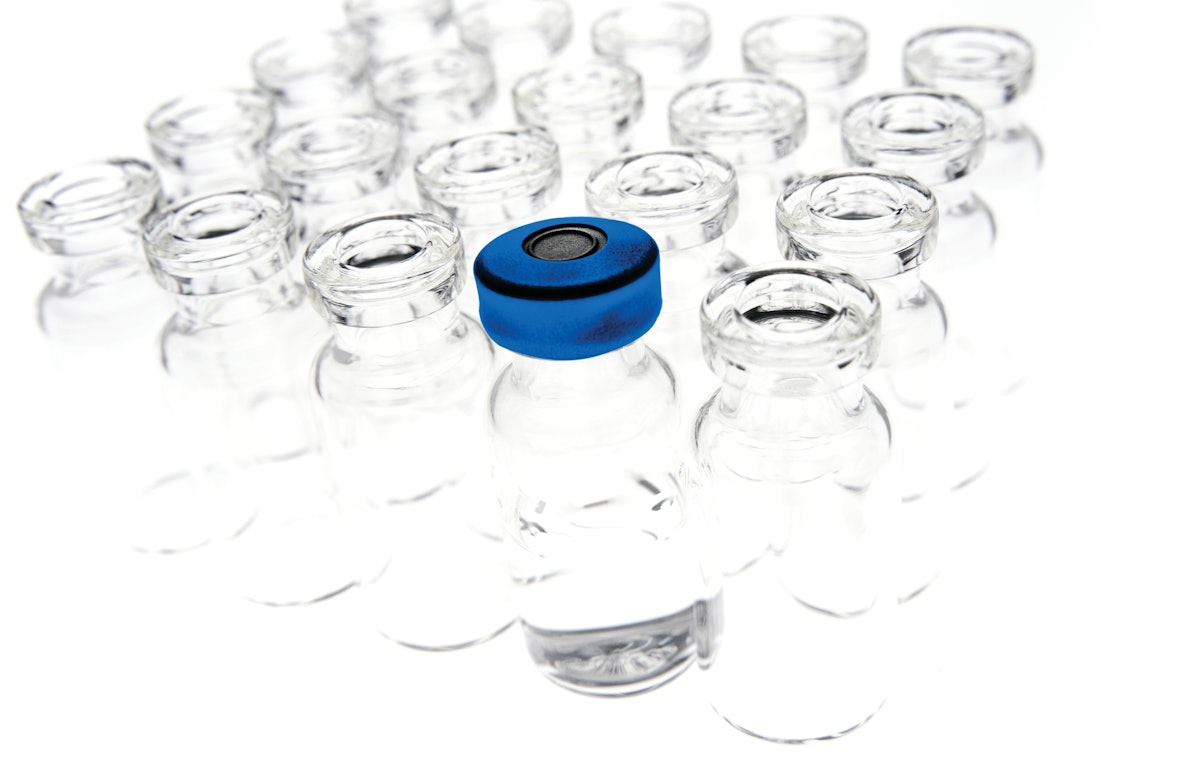
Table of Contents
Challenges in Image Acquisition for AVILDP
Consistent and reliable image acquisition is the cornerstone of successful AVILDP. However, the inherent variability of lyophilized products presents significant challenges. The process of freeze-drying creates products with diverse appearances, making automated inspection difficult.
Variations in Lyophilized Product Appearance
Lyophilized cakes exhibit considerable heterogeneity in appearance. This variability impacts the ability to acquire consistent and reliable images for analysis. Key challenges include:
-
Difficulties in standardizing lighting conditions: Shadows and reflections can significantly impact image quality, obscuring defects or creating false positives. Careful control of lighting parameters, including intensity, angle, and type, is crucial. Solutions involve advanced lighting techniques such as diffused lighting or multiple light sources.
-
Variations in cake size and shape: The size and shape of lyophilized cakes often vary, making it difficult to standardize image acquisition parameters. This necessitates adaptable imaging systems capable of adjusting to different product dimensions and orientations. Automated robotic systems capable of precise positioning and handling can mitigate this issue.
-
The need for high-resolution imaging: Subtle defects, such as micro-cracks or minute discolorations, often require high-resolution imaging for detection. The selection of appropriate camera sensors and image processing techniques is paramount in capturing these subtle details.
Dealing with Translucent and Opaque Materials
The optical properties of lyophilized products further complicate image acquisition. Some products are translucent, while others are opaque, requiring different imaging approaches.
-
Need for advanced imaging techniques: Multispectral imaging and hyperspectral imaging offer significant advantages for analyzing translucent and opaque materials. These techniques capture images across a broader range of wavelengths, allowing for better visualization of internal structures and defects.
-
Calibration challenges: Accurate calibration is essential to compensate for variations in light transmission and reflection properties of different lyophilized products. This requires sophisticated calibration protocols and algorithms that can adapt to varying material properties.
-
Development of robust algorithms: Advanced algorithms are necessary to process images obtained from diverse materials. These algorithms should be robust to noise and variations in lighting and material properties, accurately identifying defects irrespective of material characteristics.
Developing Robust Algorithms for Defect Detection in AVILDP
Even with high-quality images, robust algorithms are essential for accurate defect detection. The development of such algorithms presents its own set of challenges.
Algorithm Training and Data Requirements
Training effective machine learning algorithms for defect detection requires large, high-quality datasets representing a broad range of product variations and defect types.
-
The need for augmented reality techniques: Augmented reality (AR) can be used to create synthetic data, supplementing real-world data and enhancing the training dataset's diversity and size. This is particularly valuable for rare defects.
-
Addressing data imbalance issues: Some defect types are inherently rare, leading to imbalanced datasets that can bias algorithm performance. Techniques like data augmentation, oversampling, or cost-sensitive learning can address this issue.
-
Development of transfer learning models: Transfer learning can significantly reduce the need for large, labeled datasets. Pre-trained models can be adapted to AVILDP applications, accelerating development and improving accuracy.
Differentiating Defects from Normal Variations
Distinguishing between acceptable variations and genuine defects is a crucial challenge. Algorithms must be capable of nuanced analysis to avoid false positives and false negatives.
-
Use of advanced image processing techniques: Advanced techniques, including texture analysis, feature extraction, and fractal analysis, are invaluable for characterizing subtle variations in texture and appearance, enabling more accurate defect detection.
-
Implementation of multi-stage classification systems: Combining multiple classification algorithms in a cascade can improve accuracy and robustness. This allows for a more thorough assessment of potential defects.
-
Integration of domain expertise: Incorporating the knowledge and expertise of pharmaceutical scientists and quality control experts is crucial in refining algorithms and ensuring their accuracy and reliability.
Integration and Validation of AVILDP Systems
The successful implementation of AVILDP requires seamless integration with existing production lines and rigorous validation to meet regulatory requirements.
Integration with Existing Production Lines
AVILDP systems must be integrated smoothly into existing manufacturing workflows to avoid disrupting production and maximize efficiency.
-
Considerations for system compatibility: Careful consideration of system compatibility with existing equipment and software is vital. This includes factors such as data transfer protocols, communication interfaces, and safety standards.
-
Workflow optimization: Careful optimization of workflows is necessary to minimize downtime and maximize throughput. This often involves adjustments to existing production processes and worker training.
-
Data management: Effective data management is essential for tracking inspection results, managing quality control data, and ensuring compliance with regulatory requirements. Integration with existing quality control systems is crucial.
Validation and Regulatory Compliance
Validation is paramount to ensure the accuracy, reliability, and regulatory compliance of AVILDP systems.
-
Establishing comprehensive validation protocols: Rigorous validation protocols must be developed and followed, encompassing aspects such as system accuracy, precision, and robustness.
-
Demonstrating system accuracy and reliability: Comprehensive testing is essential to demonstrate that the system can accurately and reliably detect defects while minimizing false positives and false negatives.
-
Compliance with GMP guidelines: AVILDP systems must comply with all relevant Good Manufacturing Practices (GMP) guidelines to ensure the quality and safety of lyophilized drug products.
Conclusion
Automated visual inspection of lyophilized drug products offers significant advantages over manual inspection in terms of efficiency, consistency, and product quality. However, overcoming the challenges associated with image acquisition, algorithm development, and system integration requires a multi-faceted approach incorporating advanced imaging techniques, robust machine learning algorithms, and careful integration into existing workflows. By addressing the hurdles outlined in this article, the pharmaceutical industry can pave the way for widespread adoption of AVILDP, ultimately enhancing the safety and reliability of lyophilized drug products. To learn more about optimizing your automated visual inspection processes and leveraging the benefits of AVILDP, explore further resources on [link to relevant resource/product]. The future of quality control in lyophilized drug manufacturing lies in the advancement and implementation of efficient and reliable automated visual inspection of lyophilized drug products.
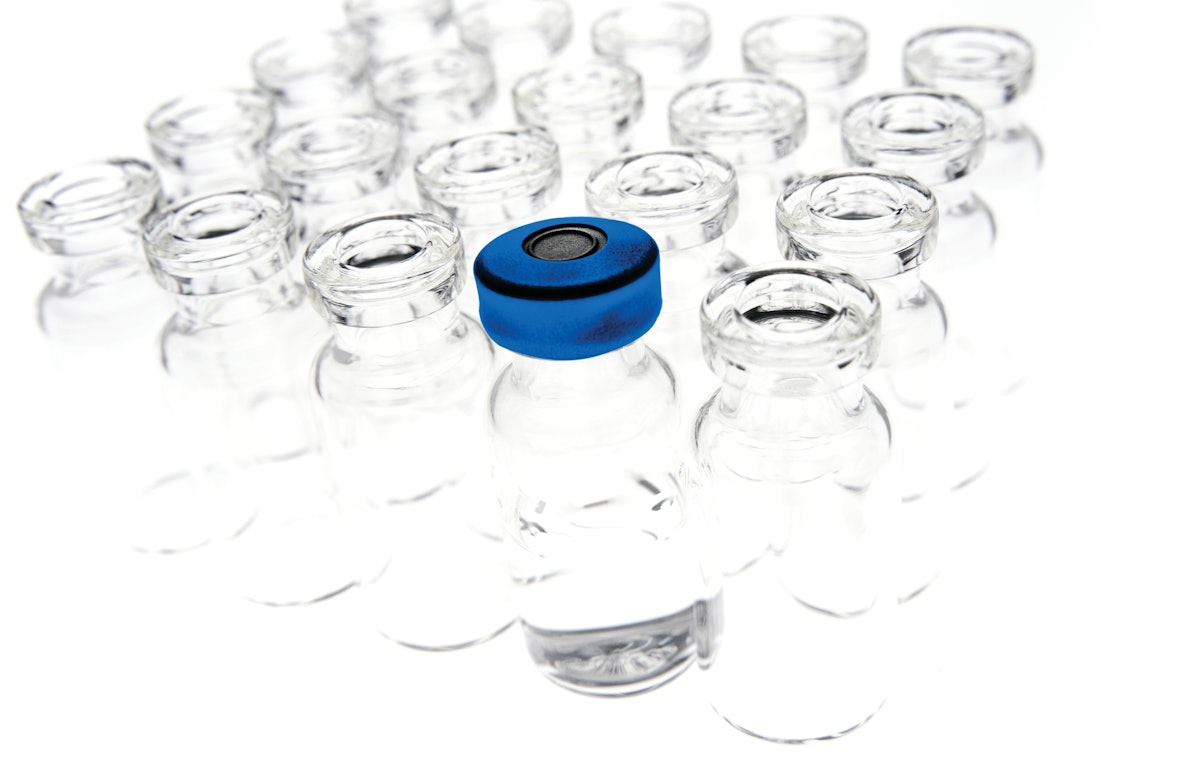
Featured Posts
-
Conclave 2023 Potential Papal Successors Among The Cardinals
May 11, 2025 -
Refugee Outings Cancelled Fabers New Policy
May 11, 2025 -
Understanding Jurickson Profars 80 Game Ped Suspension
May 11, 2025 -
Bank Of Canada Interest Rate Outlook Job Losses And The Potential For Further Cuts
May 11, 2025 -
Chantal Ladesou Actualites Et Projets A Venir
May 11, 2025