Outdated Software: A Barrier To Successful AI Implementation
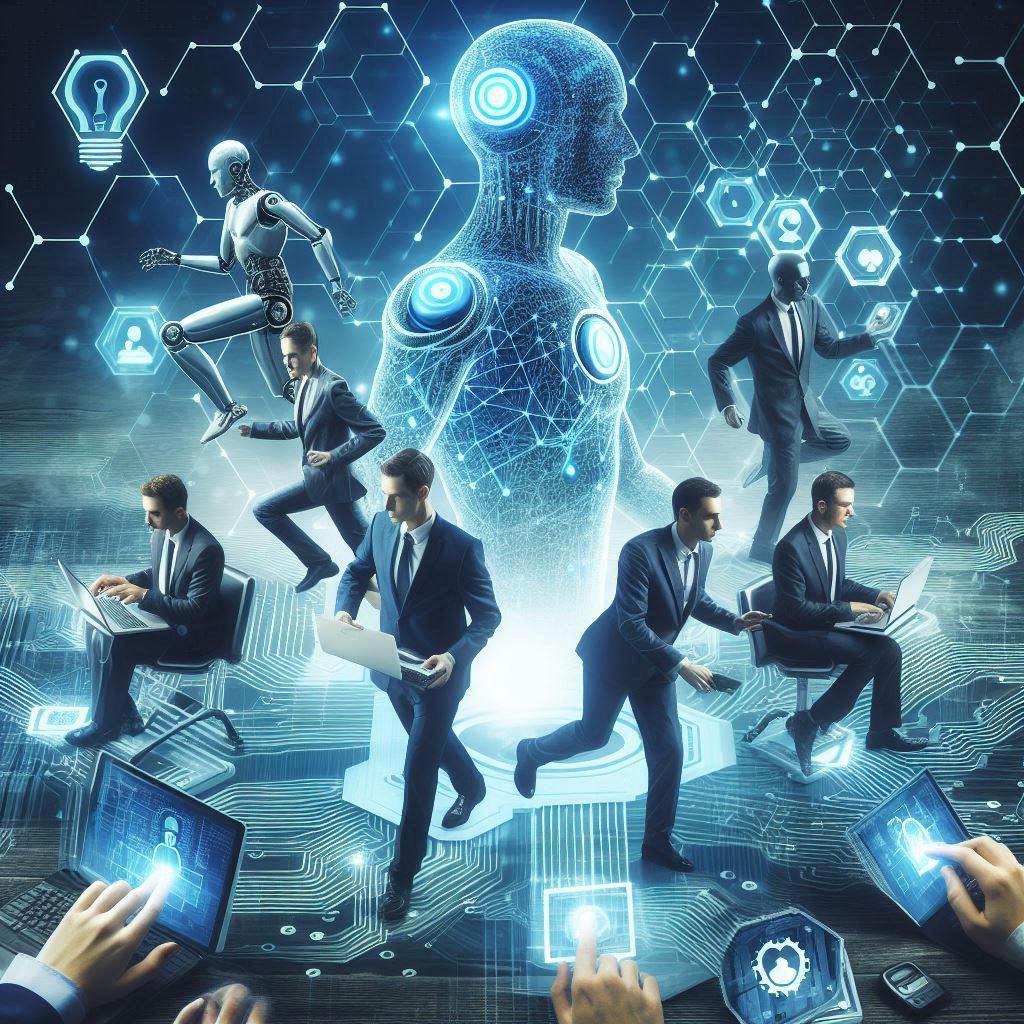
Table of Contents
Incompatibility Issues with Modern AI Technologies
The seamless integration of AI requires robust and compatible software infrastructure. Outdated software often presents significant incompatibility issues, creating a major bottleneck in the AI implementation process. Several key challenges arise from this incompatibility:
-
AI compatibility: Older systems frequently lack the necessary APIs (Application Programming Interfaces) and interfaces needed for smooth integration with modern AI tools and platforms. This lack of compatibility makes connecting AI models and existing workflows incredibly difficult, requiring extensive custom development or, in some cases, making integration impossible.
-
Software integration challenges: Successfully integrating AI often involves transferring data between various systems. With outdated software, data migration from legacy systems to AI-compatible databases can be incredibly complex, time-consuming, and prone to errors. This process requires careful planning, specialized expertise, and significant resources.
-
Scalability limitations: Modern AI often relies on processing massive datasets. Legacy systems, designed for a different era, frequently lack the scalability necessary to handle the volume and velocity of data required for effective AI model training and deployment. This limitation severely restricts the potential of AI applications.
-
Security vulnerabilities: Outdated software is notorious for security vulnerabilities, posing significant risks to data integrity. This compromise not only impacts the organization's overall security posture but also directly affects AI model accuracy and reliability, leading to flawed predictions and potentially disastrous outcomes.
Lack of Data Accessibility and Interoperability
AI thrives on data. However, outdated software frequently creates significant roadblocks to accessing and utilizing the data necessary for effective AI model development.
-
Data silos: Legacy systems often result in data silos, preventing the consolidation of information needed for robust AI applications. Data scattered across different, incompatible systems makes comprehensive analysis incredibly difficult and prevents the development of truly insightful AI models.
-
Data integration and interoperability: The lack of interoperability between different systems is another significant hurdle. AI applications frequently require access to diverse data sources, and outdated systems often lack the mechanisms to seamlessly integrate and share this information.
-
Data quality issues: Poor data quality in legacy systems is a major concern. Inaccurate, incomplete, or inconsistent data will lead directly to inaccurate AI models and flawed predictions, undermining the entire AI initiative.
-
Real-time data access: Many AI applications, especially in areas like predictive maintenance or fraud detection, require access to real-time data streams. Outdated systems often lack the capability to capture and process this information effectively, severely limiting their potential.
Increased Operational Costs and Reduced Efficiency
Implementing AI with outdated software isn’t just technically challenging; it's also significantly more expensive and less efficient.
-
Maintenance costs: Maintaining outdated software incurs substantial ongoing costs, including patches, updates, and troubleshooting. These costs can quickly escalate, draining resources that could be better invested in AI development and implementation.
-
IT infrastructure costs: Legacy systems often require specialized and expensive IT support, further impacting overall operational efficiency and adding to the overall cost of the project.
-
Reduced ROI: The complexities of integrating AI with outdated systems lead to extended project timelines and increased implementation costs, ultimately reducing the return on investment (ROI) of the AI initiative.
-
Limited automation: Modern AI solutions are designed to automate processes, boosting efficiency. However, this automation is severely limited or impossible when dealing with inflexible, outdated systems, negating a major advantage of AI implementation.
Strategies for Overcoming the Challenges of Outdated Software in AI Implementation
Addressing the challenges posed by outdated software requires a strategic and proactive approach. Several key strategies can pave the way for successful AI integration:
-
Phased approach to software modernization: Instead of attempting a complete overhaul at once, a phased approach is often more practical and less disruptive. Prioritize the modernization of critical systems directly impacting AI implementation.
-
Cloud migration: Migrating to cloud-based solutions provides access to scalable and AI-ready infrastructure, reducing many of the compatibility and scalability issues associated with legacy systems.
-
Data integration and interoperability solutions: Invest in data integration and interoperability solutions to bridge the gaps between legacy and modern systems. This allows for the consolidation and access of data critical for AI development.
-
Digital transformation strategy: Develop a comprehensive digital transformation strategy that views software modernization as an integral component of successful AI implementation. This holistic approach ensures long-term compatibility and scalability.
Conclusion
Outdated software presents significant challenges to successful AI implementation, impacting compatibility, data accessibility, operational costs, and overall efficiency. Addressing these challenges requires a strategic approach, encompassing software modernization, cloud migration, and a comprehensive digital transformation strategy. Don't let outdated software hinder your AI journey. Assess your current IT infrastructure and plan for a strategic upgrade to ensure seamless AI integration and unlock the full potential of artificial intelligence. Start your journey toward successful AI implementation by evaluating your software today!
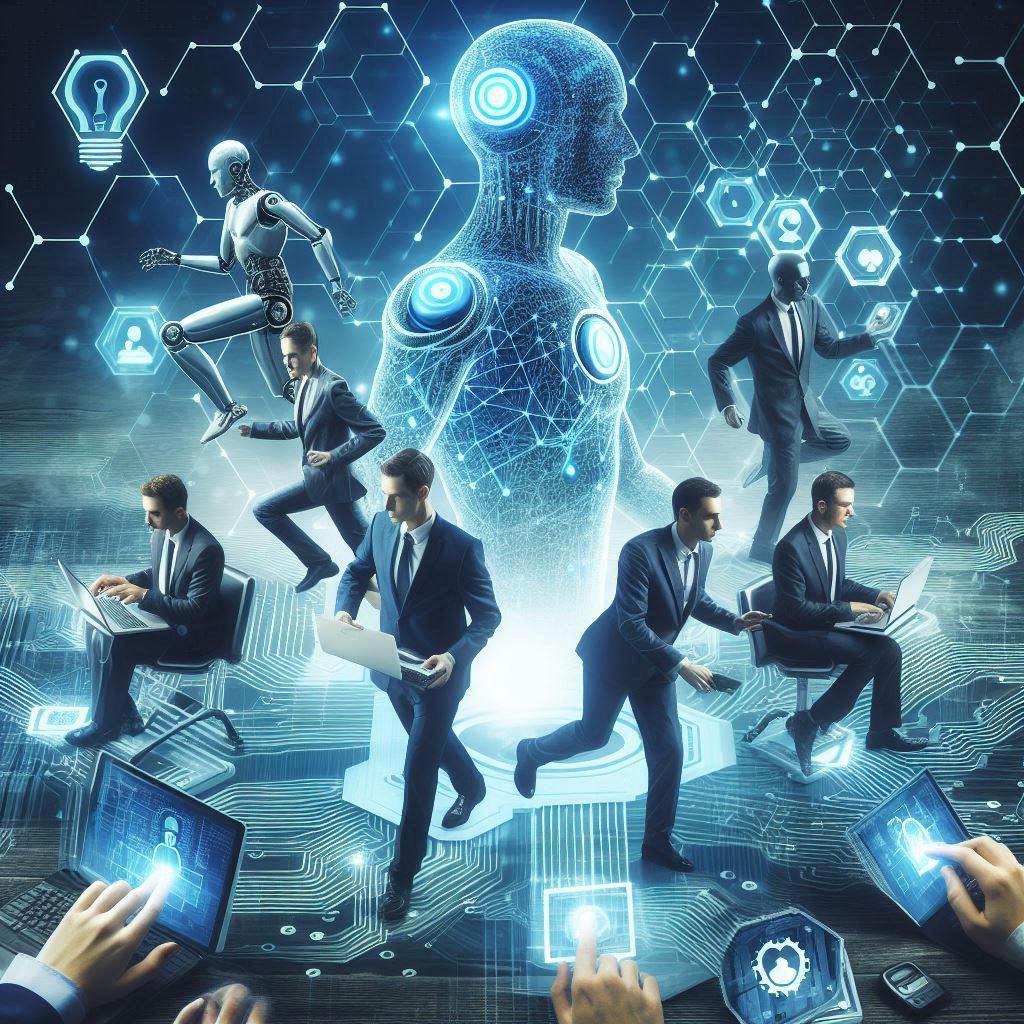
Featured Posts
-
The China Market Headwinds For Bmw Porsche And Other Premium Automakers
May 01, 2025 -
Eurovision In Manchester Dates Venue And What To Expect
May 01, 2025 -
Frances Six Nations Triumph Ramos Masterclass Against Scotland
May 01, 2025 -
The Shifting Alliance Examining The Relationship Between Altman And Nadella In The Ai Landscape
May 01, 2025 -
Bangladesh Nrc Seeks Accountability For Anti Muslim Plots
May 01, 2025
Latest Posts
-
Inisiatif Tabung Baitulmal Sarawak 125 Pelajar Asnaf Sibu Terima Bantuan Kembali Ke Sekolah 2025
May 02, 2025 -
Wachtlijsten Tbs De Gevolgen Van Overvolle Klinieken
May 02, 2025 -
Star Wars Collectible Hasbros Dash Rendar Figure From Shadow Of The Empire
May 02, 2025 -
Tbs Zorg Onder Druk Onacceptabel Lange Wachttijden Voor Patienten
May 02, 2025 -
Hasbro Debuts Highly Anticipated Dash Rendar Figure From Star Wars Shadow Of The Empire
May 02, 2025