Outdated Business Apps: Obstacles To Your AI Strategy
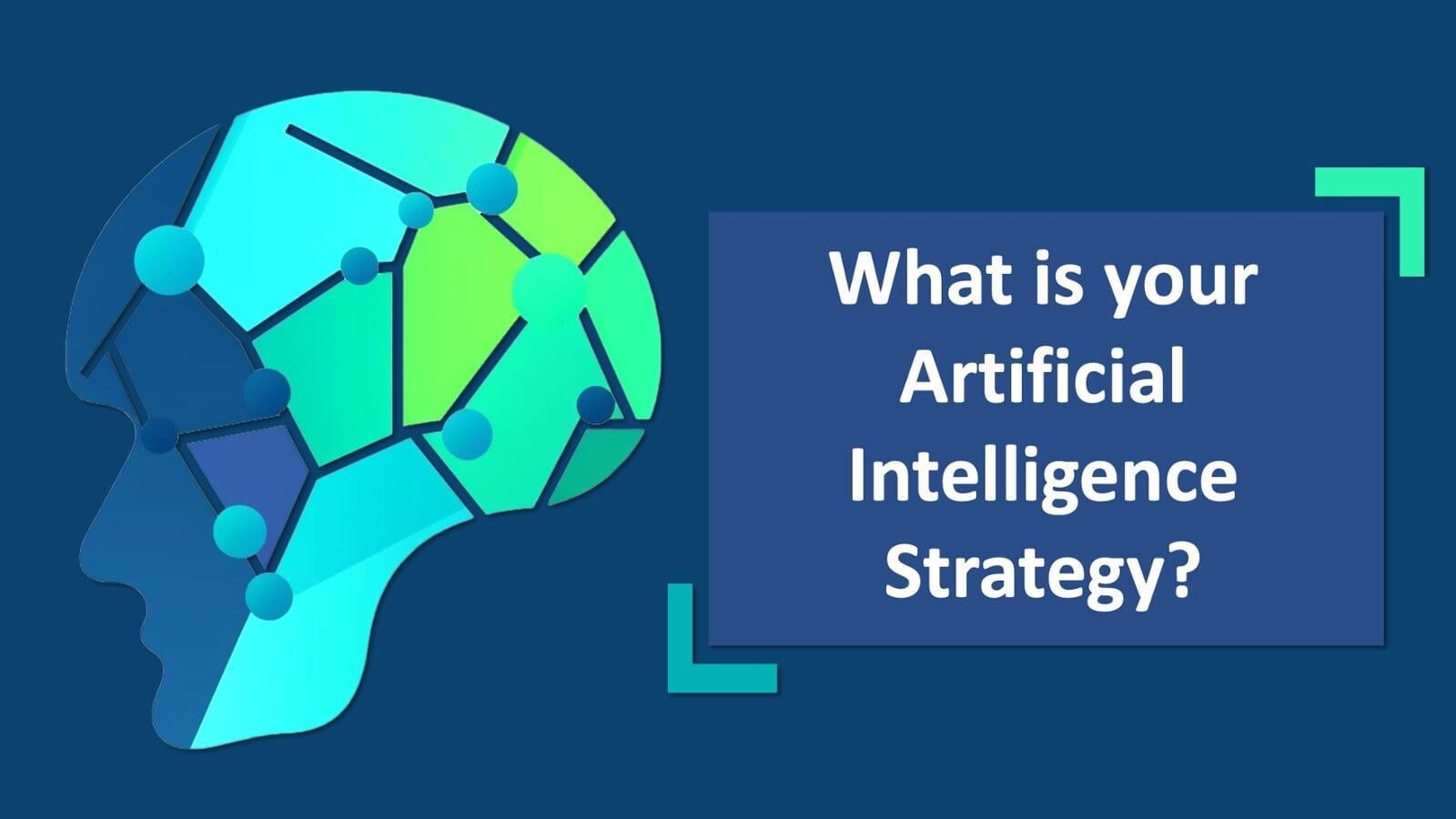
Table of Contents
Data Incompatibility and Silos: A Major AI Roadblock
Incompatible data formats and siloed data sources from older applications represent a significant roadblock to effective AI integration. AI thrives on large, clean, and consistent datasets. Outdated systems often store data in disparate formats, making consolidation for AI model training a Herculean task. This data fragmentation creates significant challenges.
-
Difficulty in consolidating data for AI model training: Trying to train an AI model on data spread across multiple incompatible systems—some using outdated databases, others relying on spreadsheets, and still others trapped in proprietary formats—is extremely difficult and time-consuming.
-
Inconsistent data quality leading to inaccurate AI predictions: Inconsistent data quality across various sources results in inaccurate AI predictions. Outdated systems often lack data validation mechanisms, leading to errors that propagate through the entire AI pipeline.
-
High costs associated with data cleaning and transformation: Before AI models can be trained, significant resources must be dedicated to cleaning and transforming data from various legacy systems. This involves significant costs in terms of time, personnel, and specialized software.
-
Lack of a unified view of customer data, hindering personalized AI experiences: For businesses relying on AI for personalized customer experiences (recommendations, targeted marketing, etc.), the lack of a unified view of customer data across different legacy systems is a major setback. This prevents the creation of a comprehensive customer profile that's crucial for personalized AI applications.
Keyword Optimization: Data integration, data silos, data compatibility, legacy systems, AI data, data quality, data consolidation, data validation.
Limited Scalability and Performance: The Bottleneck Effect
The limited scalability and processing power of older applications significantly impact AI model deployment and performance. AI models, especially deep learning models, require substantial computational resources for training and inference. Outdated systems often struggle to keep up.
-
Inability to handle the large datasets required for effective AI: Many AI algorithms require massive datasets for effective training. Legacy systems, with their limited storage capacity and processing power, might struggle to handle such datasets, hindering the development and deployment of high-performing AI models.
-
Slow processing speeds impacting real-time AI applications: Real-time AI applications, such as fraud detection or real-time customer service chatbots, demand fast processing speeds. Outdated systems, with their slow response times, can render these applications ineffective.
-
Increased infrastructure costs due to inefficient resource utilization: Legacy systems are often inefficient in their use of computing resources, leading to increased infrastructure costs to compensate for their limitations. Modern, scalable solutions often offer better resource utilization.
-
Difficulty in scaling AI solutions to meet growing business needs: As a business grows, its AI needs will also grow. Outdated systems that cannot be easily scaled might create significant bottlenecks, hindering the expansion of AI capabilities.
Keyword Optimization: Scalability, performance, AI model deployment, legacy application performance, resource utilization, real-time AI, deep learning.
Security Risks and Compliance Issues: A Threat to AI Integrity
Outdated business applications present significant security vulnerabilities and compliance issues that directly impact AI initiatives. These systems often lack modern security features and may not comply with current data protection regulations.
-
Increased risk of data breaches and security vulnerabilities: Legacy systems are often more susceptible to cyberattacks due to outdated security protocols and lack of regular updates. A data breach in a legacy system can severely compromise the integrity of AI models trained on that data.
-
Difficulty in meeting industry-specific compliance regulations (e.g., GDPR): Many industries are subject to strict data protection regulations, such as GDPR. Outdated systems may not have the necessary features to comply with these regulations, resulting in significant legal and financial risks.
-
Lack of robust security features hindering AI system protection: AI systems themselves are vulnerable to attacks. When integrated with outdated, insecure systems, the entire setup becomes a larger target for malicious actors.
-
Potential for legal and financial repercussions due to data breaches: Data breaches resulting from insecure legacy systems can lead to hefty fines, legal battles, and reputational damage.
Keyword Optimization: Data security, compliance, AI security, GDPR, data breaches, legacy system vulnerabilities, data protection regulations, cyber security.
Integration Challenges with Modern AI Tools
The lack of APIs or outdated integration capabilities in legacy applications makes connecting with modern AI platforms a significant challenge. This interoperability issue can severely impede the adoption of advanced AI tools.
-
Complex and costly custom integration projects: Integrating legacy systems with modern AI platforms often requires complex and costly custom integration projects, demanding specialized skills and resources.
-
Time-consuming data migration processes: Moving data from outdated systems to modern AI platforms can be a time-consuming and error-prone process, delaying the deployment of AI solutions.
-
Difficulty in leveraging advanced AI features: Legacy systems may not support the advanced features offered by modern AI platforms, limiting the potential benefits of AI adoption.
-
Increased risk of integration failures: The complexity of integrating legacy systems with modern AI platforms increases the risk of integration failures, potentially leading to project delays and cost overruns.
Keyword Optimization: API integration, AI platform integration, legacy system integration, data migration, AI tool integration, interoperability.
Strategies for Modernization and Mitigation
Addressing the challenges posed by outdated business applications requires a strategic approach. Here are some mitigation strategies:
-
Cloud migration to improve scalability and performance: Moving data and applications to the cloud offers improved scalability, performance, and security. Cloud-based solutions are often designed to handle the large datasets and processing demands of AI.
-
Gradual modernization of individual applications: A phased approach, focusing on modernizing individual applications based on priority and impact, can be more manageable and less disruptive than a complete overhaul.
-
Adoption of cloud-based AI solutions with pre-built integrations: Many cloud providers offer AI solutions with pre-built integrations, simplifying the integration process and reducing the need for custom development.
-
Investment in data integration tools and platforms: Investing in robust data integration tools and platforms helps streamline the process of consolidating data from disparate sources, improving data quality and enabling seamless AI integration.
Keyword Optimization: Application modernization, cloud migration, AI solutions, data integration tools, legacy system replacement, phased approach, cloud-based AI.
Conclusion
Outdated business applications pose significant obstacles to a successful AI strategy. Addressing data incompatibility, scalability issues, security risks, and integration challenges is crucial for maximizing the benefits of AI. By strategically modernizing legacy systems and adopting a phased approach to AI integration, businesses can unlock the full potential of artificial intelligence and gain a competitive edge. Don't let your outdated business apps hinder your AI strategy – start planning your modernization journey today!
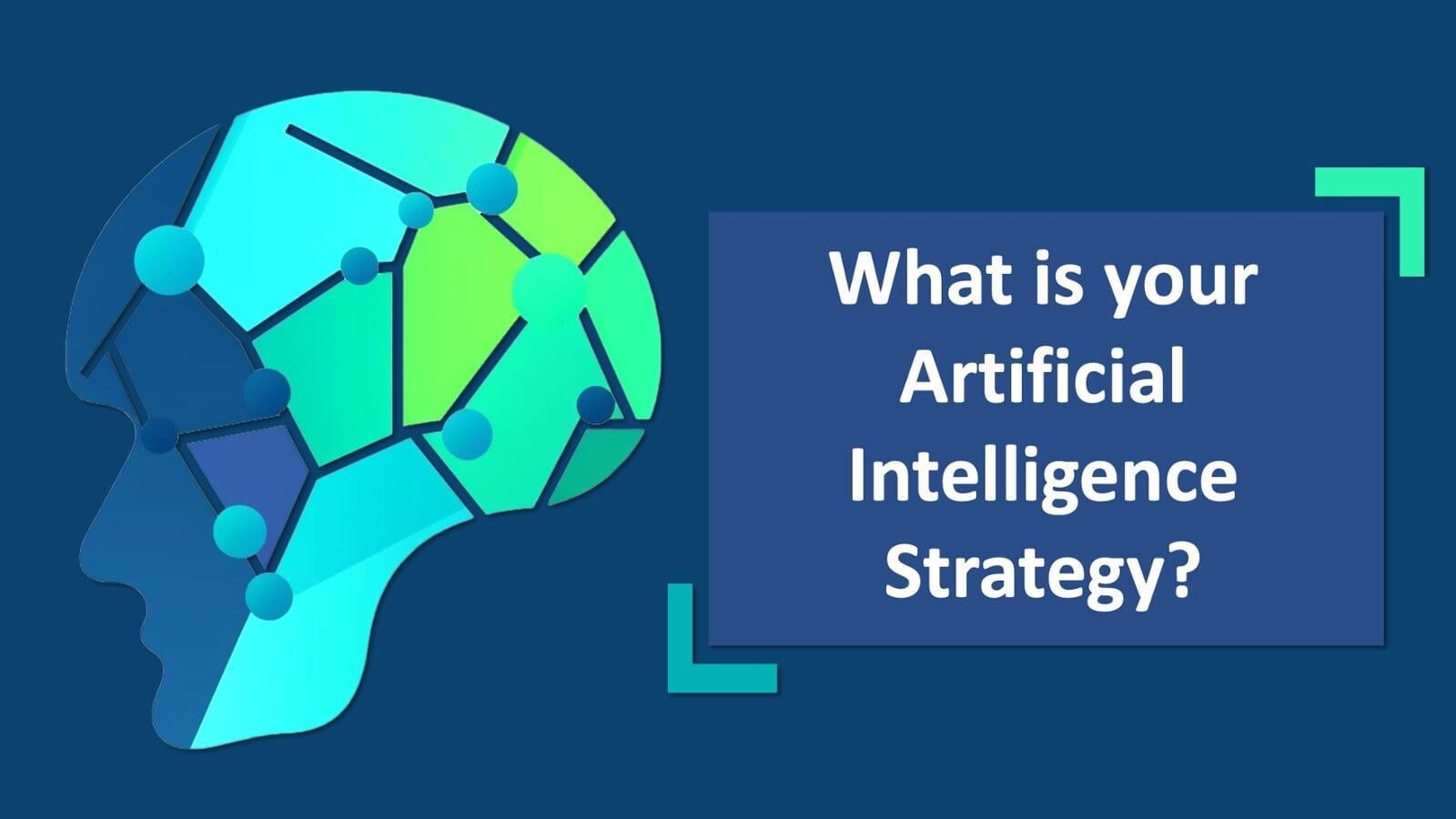
Featured Posts
-
Acces Au Document Amf Cp 2025 E1029244 D Edenred
Apr 30, 2025 -
Lca
Apr 30, 2025 -
Zelenskiy I Tramp Veroyatnost Vstrechi Na Pokhoronakh Papy Rimskogo
Apr 30, 2025 -
Chris Paul Harrison Barnes And Julian Champagnie A Look At Their Spurs Game Attendance This Season
Apr 30, 2025 -
Analisi Delle Chat Pubblicate Da Domani Il Ruolo Di Becciu Nel Complotto
Apr 30, 2025
Latest Posts
-
Police Complaints Body Targets Chris Kaba Panorama Ofcom Intervention Sought
Apr 30, 2025 -
Police Watchdogs Ofcom Complaint Chris Kaba Panorama Episode
Apr 30, 2025 -
Chris Kaba Shooting Police Complaint Leads To Ofcom Review Of Bbc Panorama
Apr 30, 2025 -
Independent Office For Police Conduct Iopc Challenges Bbcs Chris Kaba Panorama
Apr 30, 2025 -
Panorama Under Fire Police Watchdogs Formal Ofcom Complaint Over Chris Kaba Coverage
Apr 30, 2025