Is Your Old Software Holding Back Your AI Goals?
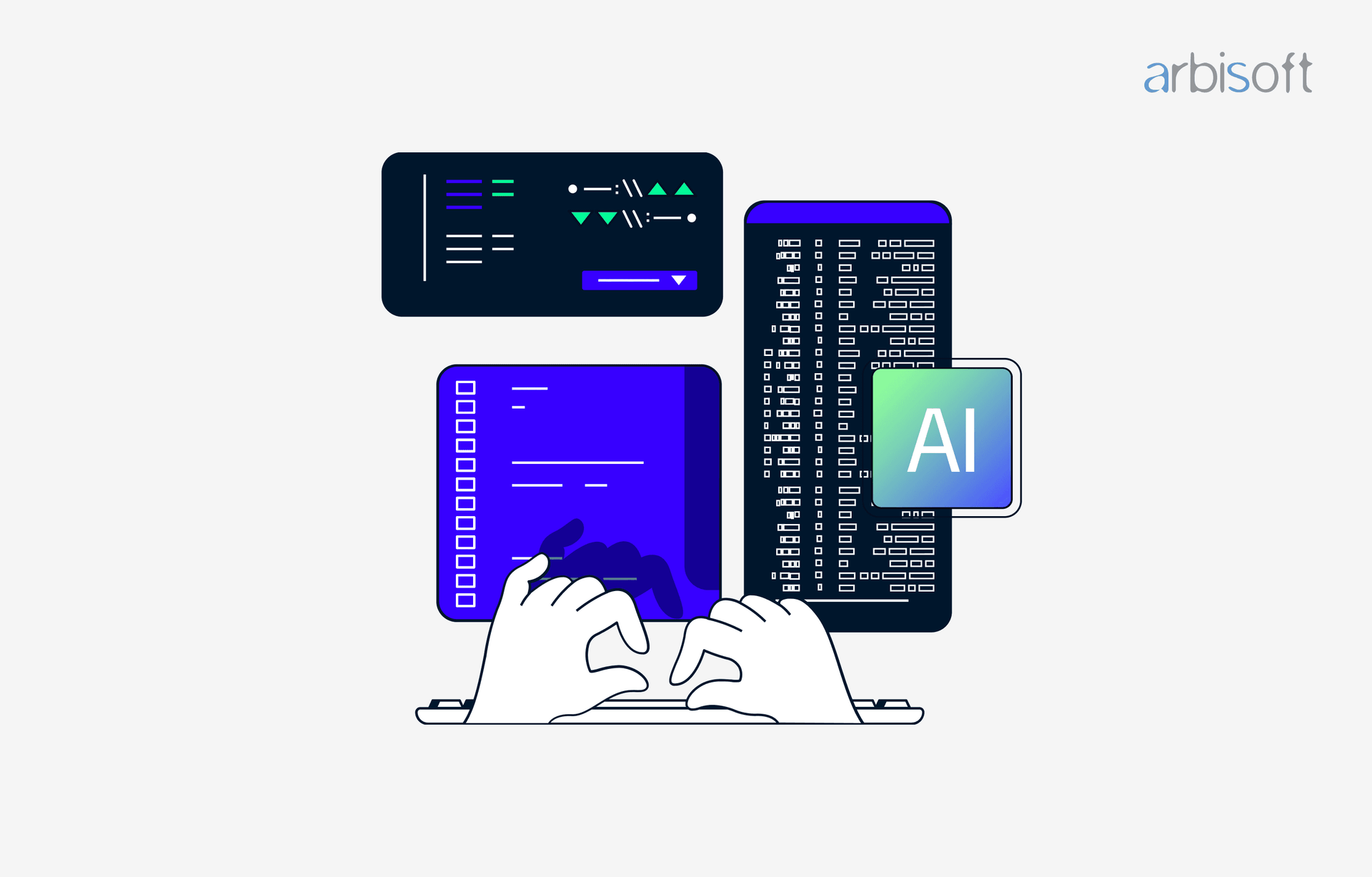
Table of Contents
Data Compatibility Issues with Legacy Systems
Legacy systems, with their disparate data sources and antiquated architecture, present significant hurdles to successful AI implementation. The data required to train robust and accurate AI models needs to be comprehensive, consistent, and readily accessible. Old software frequently fails to meet these requirements.
Data Silos and Integration Challenges
Many older systems create data silos, making it extremely difficult to build a cohesive view of your data. This leads to several critical issues:
- Difficulty accessing data: Data might be trapped in various incompatible formats across different departments or systems.
- Inconsistent data formats: Data inconsistencies hamper the training of AI models, leading to inaccurate predictions and unreliable outcomes.
- Lack of APIs: The absence of Application Programming Interfaces (APIs) makes it challenging to integrate data from legacy systems into modern AI platforms.
- High integration costs: Connecting disparate data sources requires significant time, effort, and financial resources, often exceeding initial project budgets.
For example, a retail company struggling with separate inventory management, customer relationship management (CRM), and sales data systems found it nearly impossible to build a predictive AI model for demand forecasting. The lack of integrated data resulted in significant inaccuracies and ultimately hindered sales optimization.
Data Security and Privacy Concerns with Outdated Software
Outdated software poses significant security risks, jeopardizing the sensitive data crucial for AI development. This also creates compliance issues related to regulations like GDPR and CCPA.
- Lack of encryption: Older systems may lack robust encryption protocols, leaving sensitive data vulnerable to breaches.
- Outdated security protocols: Legacy systems often fail to meet modern security standards, increasing the risk of cyberattacks and data theft.
- Difficulty in auditing data access: Tracking and auditing data access in outdated systems can be challenging, making it difficult to ensure compliance and identify potential security vulnerabilities.
A recent case study highlighted a healthcare provider whose legacy system suffered a data breach, compromising patient medical records used in an ongoing AI-driven diagnostic project. This not only resulted in significant financial penalties but also severely damaged the provider’s reputation.
Scalability and Performance Limitations
AI models, particularly deep learning models, require substantial computing power and resources to process large datasets and complex algorithms. Legacy systems, often built for smaller-scale operations, frequently lack this capacity.
Processing Power and Resource Constraints
Older systems often face the following limitations:
- Slow processing speeds: Processing large datasets can take an excessively long time, hindering the efficiency and effectiveness of AI development.
- Limited memory capacity: Insufficient memory limits the size and complexity of the AI models that can be trained.
- Inability to scale to meet growing data demands: As data volumes increase, legacy systems may struggle to keep up, leading to performance bottlenecks.
For instance, comparing the processing time of a facial recognition AI model running on a legacy system versus a modern cloud-based solution reveals a stark difference. The cloud-based solution processes data orders of magnitude faster, enabling real-time analysis and significantly improving accuracy.
Infrastructure Limitations and Maintenance Costs
Maintaining legacy systems comes with significant hidden costs that directly impact AI project timelines and budgets:
- Higher operational costs: Maintaining outdated hardware and software requires substantial ongoing investment.
- Difficulty in finding skilled personnel: Finding experts capable of maintaining obsolete systems is increasingly challenging.
- Increased risk of system failures: Older systems are more prone to malfunctions and unexpected downtime, disrupting AI development and deployment.
A cost-benefit analysis comparing the maintenance of a legacy system with a modern, cloud-based solution for AI often reveals substantial savings in the long run, outweighing the initial investment in upgrading infrastructure.
The Impact on AI Innovation and Future-Proofing
Relying on outdated software severely restricts your ability to leverage the full potential of AI and adapt to future advancements.
Limited AI Capabilities and Functionality
Outdated software can significantly limit AI capabilities:
- Incompatibility with advanced AI tools and algorithms: Legacy systems may not support cutting-edge AI tools and algorithms, hindering innovation.
- Inability to leverage cloud-based AI services: Many modern AI solutions rely on cloud-based infrastructure, which older systems may not be able to integrate with effectively.
- Reduced ability to adapt to new AI technologies: As AI continues to evolve, outdated software makes it difficult to adapt to new technologies and maintain a competitive edge.
Imagine a manufacturing company unable to adopt a new AI-powered predictive maintenance tool due to incompatibility with its existing legacy system, resulting in increased downtime and lost productivity.
Difficulty in Attracting and Retaining AI Talent
Top AI talent seeks modern, efficient tools and technologies. Outdated systems create obstacles:
- Lack of appealing work environment: Working with outdated technology can be frustrating and demotivating for skilled professionals.
- Limited opportunities for professional development: Legacy systems restrict access to the latest AI tools and techniques, hindering professional growth.
- Inability to utilize the latest AI technologies: The lack of access to modern tools may make it difficult for companies to attract and retain talented AI engineers and data scientists.
A company struggling to recruit experienced data scientists found that their outdated infrastructure was a major deterrent for potential candidates who were seeking opportunities to work with the latest technologies and methodologies.
Conclusion
Outdated software presents significant challenges to achieving your AI goals. From data compatibility issues and scalability limitations to the impact on innovation and talent acquisition, neglecting your software infrastructure can severely hinder your AI initiatives. Don't let your old software hold back your AI goals. Assess your current systems and take proactive steps towards modernization. Embrace digital transformation by upgrading your infrastructure and unlocking the true potential of AI. Investing in modern, scalable, and secure software is crucial for achieving your AI objectives and staying competitive in the rapidly evolving world of artificial intelligence.
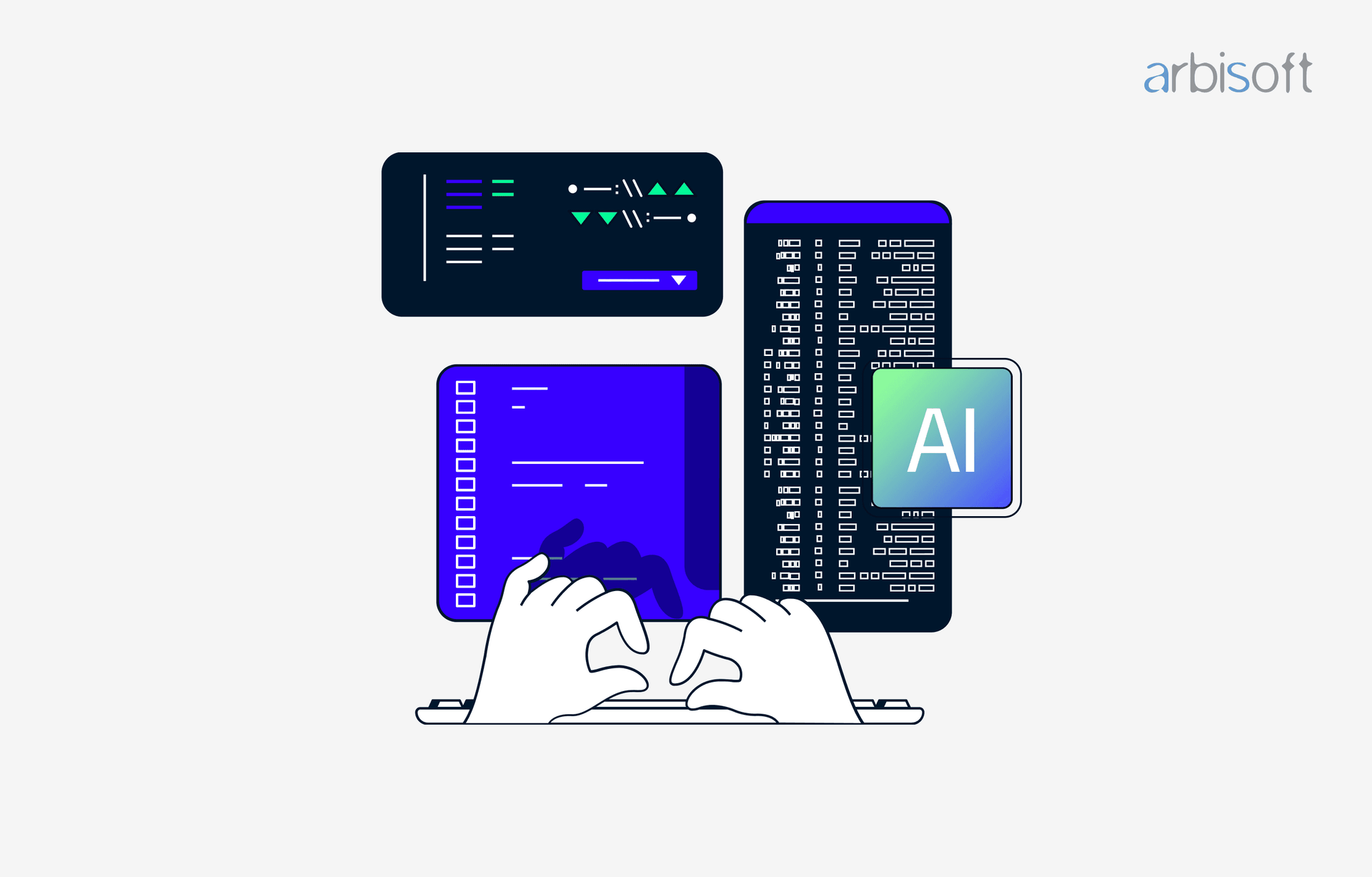
Featured Posts
-
Baitulmal Sarawak Salurkan Bantuan Rm 36 45 Juta Kepada Asnaf Mac 2025
May 01, 2025 -
Brtanwy Parlymnt Kshmyr Ke Msyle Ke Hl Ky Hmayt
May 01, 2025 -
Duponts 11 Point Contribution Decisive Factor In Frances Victory Over Italy
May 01, 2025 -
Christopher Stevens Reviews Michael Sheens Debt Charity Project
May 01, 2025 -
Enexis Wachtlijst Van Meer Dan 1000 Limburgse Ondernemers
May 01, 2025
Latest Posts
-
Stroomproblemen Nieuw Duurzaam Schoolgebouw Kampen Gemeente Start Kort Geding
May 02, 2025 -
Gemeente Kampen Vs Enexis Kort Geding Over Stroomnetaansluiting
May 02, 2025 -
Geen Stroom Voor Nieuw Duurzaam Schoolgebouw Kampen Kort Geding Gestart
May 02, 2025 -
Duurzaam Schoolgebouw Kampen Rechtszaak Tegen Stroomnetaansluiting
May 02, 2025 -
Gemeente Kampen Start Kort Geding Wegens Geweigerde Stroomnetaansluiting
May 02, 2025