AI And Wildlife Conservation: Benefits, Challenges, And The Future
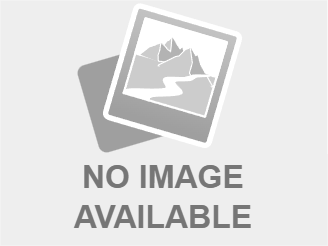
Table of Contents
Benefits of AI in Wildlife Conservation
AI is revolutionizing how we approach wildlife conservation, offering several key advantages:
Improved Monitoring and Surveillance
AI-powered surveillance is significantly enhancing our ability to monitor wildlife populations and combat illegal activities.
- Drone technology coupled with AI-powered image recognition allows for efficient tracking of endangered species across vast and often inaccessible landscapes. Drones can capture high-resolution images and videos, which AI algorithms then analyze to identify and count individual animals, assess their health, and monitor their behavior.
- Real-time monitoring enables prompt responses to threats, such as habitat encroachment or poaching activity. Systems can be set up to alert authorities immediately upon detection of suspicious movements or activities.
- Poaching detection is greatly improved through AI analysis of camera trap images. Algorithms can distinguish between animals and humans, identify weapons, and even recognize individual poachers based on their clothing or physical characteristics. This leads to more effective anti-poaching strategies.
- Examples include analyzing camera trap images to identify individual tigers based on their unique stripe patterns, monitoring elephant herds to detect signs of stress or illness, and tracking deforestation rates in real-time.
Keywords: AI-powered surveillance, wildlife monitoring, drone technology, image recognition, poaching detection
Predictive Modeling for Conservation Strategies
AI algorithms are proving invaluable for predictive modeling in conservation planning.
- Species distribution modeling uses AI to predict where endangered species are likely to be found based on environmental factors such as habitat type, climate, and prey availability. This information helps focus conservation efforts on the most crucial areas.
- Habitat modeling can predict how habitat loss and fragmentation will impact wildlife populations, allowing for proactive conservation measures to mitigate these impacts.
- Risk assessment models can identify high-risk areas for poaching, disease outbreaks, or other threats, facilitating targeted interventions.
- By simulating various conservation scenarios, AI can help optimize resource allocation and determine the most effective strategies for protecting biodiversity.
Keywords: Predictive analytics, conservation planning, habitat modeling, species distribution modeling, risk assessment
Enhanced Data Analysis and Management
The sheer volume of ecological data generated from various sources is overwhelming for manual analysis. AI offers a solution:
- AI can process vast amounts of data from satellite imagery, sensor networks, and citizen science initiatives, identifying subtle patterns and trends that humans would miss.
- Machine learning algorithms can detect anomalies and predict future trends, providing valuable insights for conservation decision-making. This includes identifying changes in habitat quality, detecting disease outbreaks early, or predicting the spread of invasive species.
- AI improves data management and accessibility, making it easier for researchers and conservationists to share and analyze data collaboratively, fostering a more comprehensive understanding of ecological systems.
- AI-powered data visualization tools can also help communicate complex scientific findings to a wider audience, engaging the public and policymakers in conservation efforts.
Keywords: Big data analytics, data management, ecological data, machine learning, data visualization
Challenges in Implementing AI for Wildlife Conservation
Despite its potential, integrating AI into wildlife conservation faces several hurdles:
Data Acquisition and Quality
The success of AI in conservation depends heavily on the availability of high-quality data.
- Data scarcity is a major challenge, particularly in remote or data-poor regions. Collecting sufficient, reliable data for training AI algorithms is often time-consuming and expensive.
- Data labelling is crucial but requires significant manual effort, involving experts identifying and classifying images, sounds, or other data points. This process is resource-intensive.
- Data biases can significantly affect the accuracy and fairness of AI algorithms. Biases can arise from various sources, such as sampling methods, data collection techniques, or the inherent limitations of existing datasets. Addressing data biases is crucial for ensuring that AI-driven conservation efforts are equitable and effective.
Keywords: Data scarcity, data labelling, data bias, remote sensing, data collection
Technological Limitations and Costs
Advanced AI algorithms require significant computational power and resources.
- High computational costs can be a barrier for many conservation organizations, particularly those with limited budgets.
- Accessibility of AI technologies to organizations in developing countries is a major concern. Technological transfer and capacity building are essential to bridge this gap.
- AI systems need to be robust and reliable, capable of functioning effectively in challenging environmental conditions, such as extreme temperatures, limited connectivity, or harsh terrains.
Keywords: Computational power, AI accessibility, cost-effectiveness, technology transfer
Ethical Considerations
The application of AI in wildlife conservation raises important ethical considerations.
- Data privacy is a key concern, especially with the use of AI-powered surveillance technologies. Strict guidelines and regulations are needed to protect the privacy of individuals and communities.
- Responsible AI development requires careful consideration of potential unintended consequences of AI interventions on wildlife behavior and ecosystems. It is crucial to evaluate potential risks and ensure that AI is used in a way that promotes wildlife welfare and ecosystem integrity.
- Ensuring ethical data collection is paramount, respecting the rights and well-being of local communities and minimizing any negative environmental impacts.
Keywords: AI ethics, data privacy, responsible AI, wildlife welfare, environmental impact
The Future of AI in Wildlife Conservation
The future of AI in wildlife conservation looks promising, with several exciting developments on the horizon:
- Advancements in AI, such as deep learning and reinforcement learning, will lead to even more accurate and efficient conservation tools.
- Increased collaboration between AI researchers, conservation biologists, and policymakers is crucial for successful implementation of AI in conservation strategies.
- The development of open-source AI platforms and tools will make AI more accessible to conservation organizations worldwide.
- Integration of AI with other technologies, such as remote sensing, drones, and citizen science platforms, will create powerful synergies for improved monitoring, data analysis, and conservation management.
Keywords: Deep learning, reinforcement learning, collaborative research, open-source AI, technology integration
Conclusion
AI offers significant benefits for wildlife conservation, from improving monitoring and surveillance to enabling predictive modeling and enhancing data analysis. However, challenges related to data acquisition, technological limitations, and ethical considerations need to be addressed proactively. The transformative potential of AI to address urgent conservation issues is undeniable. The future of wildlife conservation hinges on harnessing the power of AI. Learn more about how you can contribute to this critical field and help protect our planet's biodiversity through AI-driven conservation efforts.
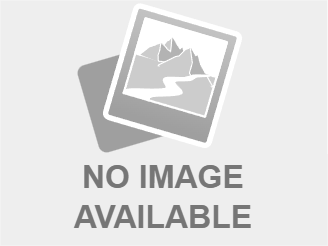
Featured Posts
-
Morning Retail Et Nutriscore Decryptage Des Pratiques Industrielles
Apr 23, 2025 -
Office365 Security Failure Millions Stolen Investigation Underway
Apr 23, 2025 -
Pentrich Brewing Company Factory Tour And Tasting Experience
Apr 23, 2025 -
Calendario Laboral 2024 El Puente Del 21 De Abril Y Sus Implicaciones
Apr 23, 2025 -
Removing Barriers To Internal Trade In Ontario Alcohol And Labour Mobility Reforms
Apr 23, 2025