Addressing Legacy Systems To Achieve AI Success
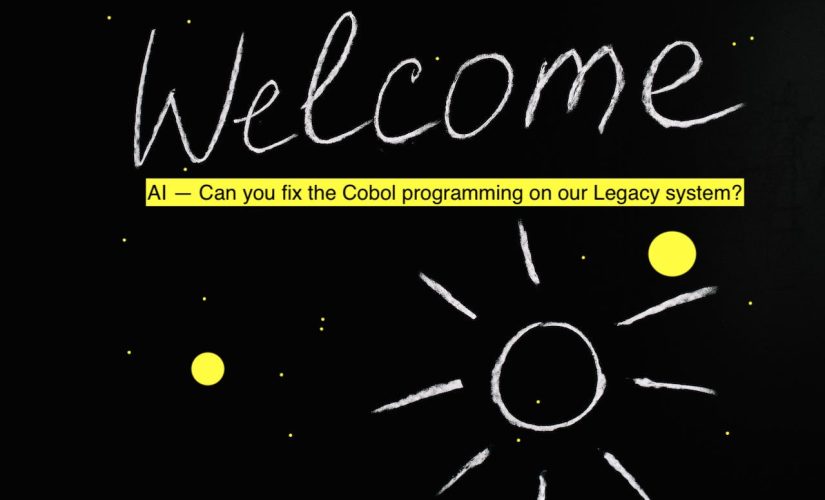
Table of Contents
Assessing Your Legacy Systems for AI Integration
Before embarking on AI integration, a thorough assessment of your legacy systems is paramount. This crucial step identifies potential roadblocks and informs the modernization strategy. Key aspects to evaluate include:
-
Data accessibility and quality: Can your AI algorithms readily access the necessary data? Is the data clean, consistent, and reliable, or does it require extensive cleaning and transformation? Legacy systems often suffer from data silos and inconsistencies, hindering AI model accuracy. Consider data profiling tools to assess data quality and identify potential issues.
-
System compatibility: Are your legacy systems compatible with modern AI tools and technologies? Do you have the necessary APIs and infrastructure to facilitate seamless data exchange? Many legacy systems lack the APIs or standardized interfaces required for efficient AI integration.
-
Scalability and performance: Can your existing infrastructure handle the increased processing demands of AI algorithms? Legacy systems, often built for specific tasks, may lack the scalability needed to process large datasets and support complex AI models. Performance bottlenecks can significantly impact AI model training and deployment times.
-
Security vulnerabilities and data governance: Legacy systems may have outdated security protocols, increasing vulnerability to cyber threats and data breaches. Compliance with regulations like GDPR and CCPA is crucial. A comprehensive security assessment is essential before integrating AI.
Tools like legacy system analysis software, database profiling tools, and API testing frameworks can significantly aid in this evaluation process.
Strategies for Modernizing Legacy Systems for AI
Modernizing legacy systems for AI integration involves several approaches, each with its own advantages and disadvantages. The optimal strategy depends on the specific system, its complexity, and business needs. Consider these options:
-
Encapsulation/Wrapping: This approach involves building a layer around the legacy system, allowing interaction with modern AI tools without requiring extensive code changes. It's a quicker solution, ideal for systems that are difficult or costly to modify directly.
-
Refactoring: This involves restructuring the existing codebase to improve its design, maintainability, and integration capabilities. It's suitable for systems that are relatively well-documented and where parts can be incrementally improved.
-
Rehosting/Migration to the Cloud: Moving the legacy system to a cloud environment offers scalability, flexibility, and access to modern AI services. However, migration can be complex and require significant upfront investment.
-
Replacement: In some cases, a complete replacement of the legacy system with a modern, AI-ready solution is the most effective approach. This is generally the most expensive option but offers the greatest long-term benefits.
Successful modernization projects often involve a hybrid approach, combining different strategies to address specific system components. For example, a company might refactor critical modules while encapsulating others before migrating to a cloud-based infrastructure.
Data Integration and Transformation for AI
Data forms the foundation of any successful AI implementation. Extracting, cleaning, and transforming data from legacy systems often presents significant challenges. These challenges include data inconsistencies, missing values, and different data formats. Effective techniques for data integration include:
-
ETL (Extract, Transform, Load) processes: These processes automate the extraction of data from various sources, transform it into a consistent format, and load it into a data warehouse or data lake suitable for AI processing.
-
API integration: Using APIs to connect legacy systems with modern AI tools allows for real-time data exchange and seamless integration.
-
Data virtualization: This approach allows access to data from different sources without physically moving or transforming it, providing a unified view for AI algorithms.
Maintaining high data quality is paramount. Inaccurate or incomplete data will lead to inaccurate AI model predictions. Implementing robust data validation and cleansing procedures is crucial for ensuring data quality.
Building and Deploying AI Solutions with Legacy Systems
Integrating AI models with existing systems often requires careful planning and execution. Effective approaches include:
-
Microservices architecture: This approach breaks down the AI solution into smaller, independent services that can be developed, deployed, and scaled independently. This improves flexibility and allows for gradual integration with legacy systems.
-
API-driven integration: Using APIs to connect AI models with legacy systems enables seamless data exchange and communication.
-
Hybrid cloud deployment strategies: Combining on-premise infrastructure with cloud-based resources allows organizations to leverage the benefits of both while addressing the constraints of legacy systems.
Potential challenges include latency, scalability issues, and security concerns. Rigorous testing and monitoring are critical to ensuring the reliability and performance of AI solutions.
Securing Your AI Systems and Data
Security is a paramount concern when integrating AI with legacy systems. Protecting sensitive data and ensuring compliance with relevant regulations are vital. Key security measures include:
-
Data encryption: Encrypting data both in transit and at rest protects it from unauthorized access.
-
Access control: Implementing robust access control mechanisms limits access to sensitive data and AI models to authorized personnel only.
-
Regular security audits: Regularly auditing your systems helps identify and address potential security vulnerabilities.
A comprehensive security framework, encompassing all stages of the AI lifecycle, is crucial for protecting your AI systems and data.
Conclusion: Achieving AI Success Through Legacy System Modernization
Successfully integrating AI with legacy systems requires a strategic and phased approach. This article highlighted the challenges involved in assessing legacy systems, choosing appropriate modernization strategies, effectively integrating data, building and deploying AI solutions, and ensuring robust security. By carefully considering these aspects, organizations can overcome the hurdles of legacy systems and unlock the transformative power of AI. To begin your AI journey and start addressing legacy systems to achieve AI success, assess your current infrastructure and explore available modernization options. Don't let legacy systems hinder your progress – embrace the future of AI today! [Link to relevant resource, e.g., a consultation or white paper].
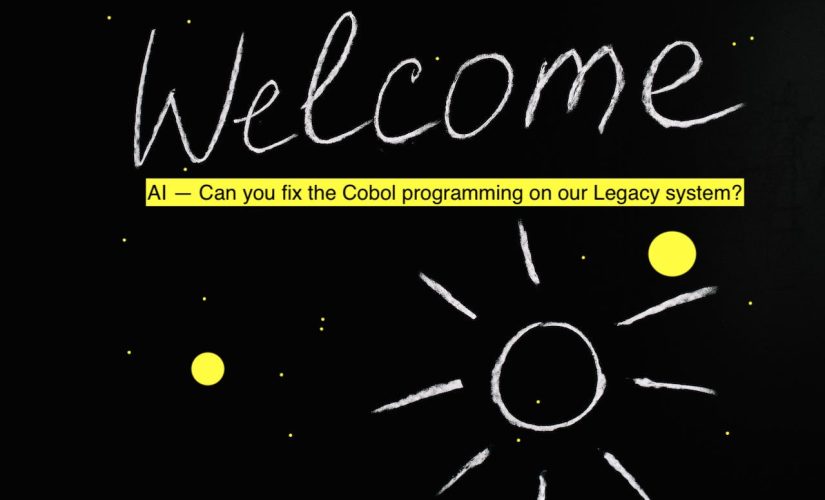
Featured Posts
-
The 51st State Debate Understanding Trumps Stance On Canada
Apr 30, 2025 -
Coronation Street Actor Speaks Out After Heartbreaking Departure
Apr 30, 2025 -
I Ygeia Mas Se Ines Pos Oi Ypologistes Tha Allaksoyn Ta Panta
Apr 30, 2025 -
Mta Ysrf Meashat Abryl 2025 Altarykh Alrsmy L 13 Mlywn Mwatn
Apr 30, 2025 -
Essential Wayne Gretzky Fast Facts For Hockey Fans
Apr 30, 2025